This content originally appeared on Level Up Coding - Medium and was authored by Julian Sitkevich
In its own words, ChatGPT introduces ChatGPT
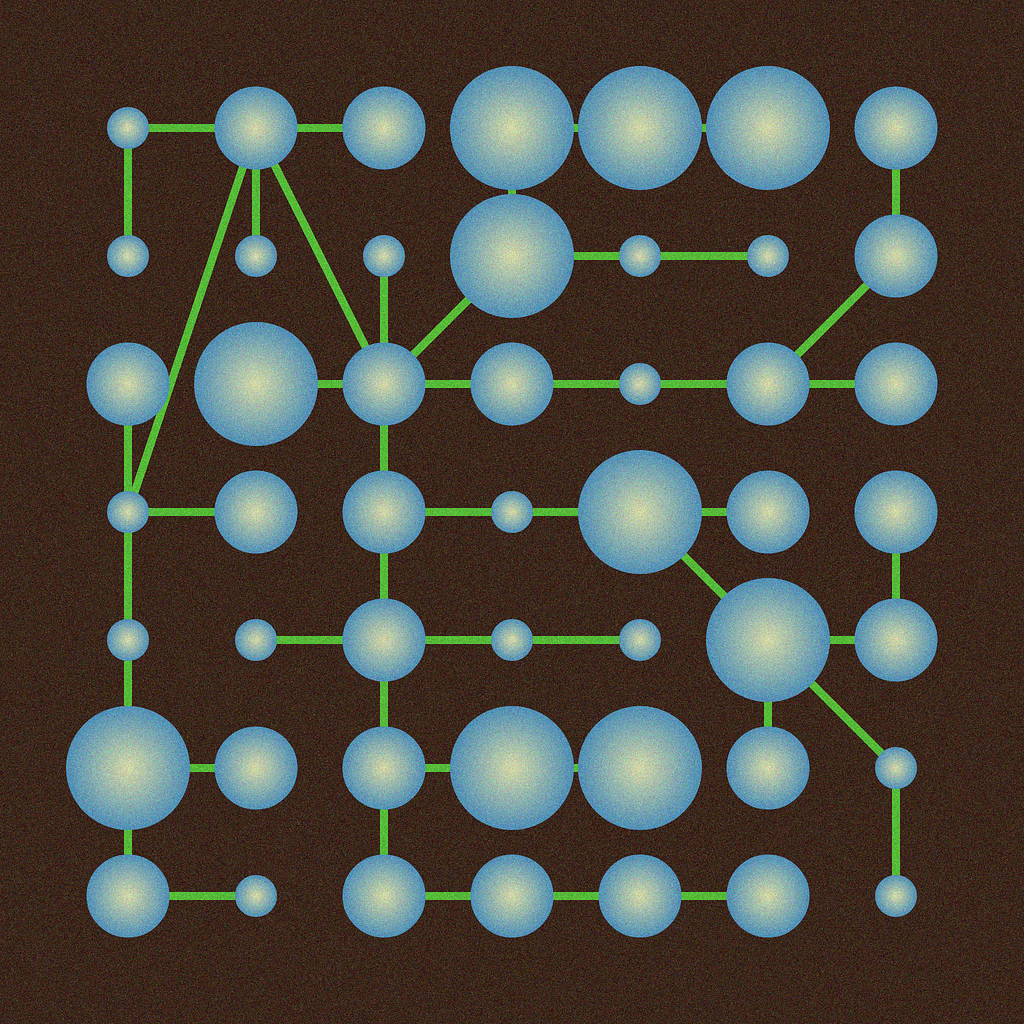
Ever since ChatGPT launched in late November 2022, it quickly created a phenomenon, taking the world by storm. Some staggering adoption metrics too, gaining one million users in a week following its launch. There’s been no shortage of opinions, with some stating ChatGPT is the most innovative technology available to the masses since the inception of the Internet, or as important as the invention of the modern smartphone. And of course some predictions on how ChatGPT will impact us as individuals, society, across industries and the economy.
So to the task at hand, let’s take this moment to interview ChatGPT itself, and hear about ChatGPT in its own words. I’ve tried to focus on questions around introductory, “how to” and basic usage with this interview. And to also shed insight on basic features, capabilities and limitations. As you’ll see, for some of the more detailed interview questions, ChatGPT doesn’t always provide a sufficient answer.
Before we get underway, I’d like to both note and share a disclaimer, that the ChatGPT generated answer responses are text generated solely from ChatGPT, this text is unedited and may not be entirely factual or accurate.
ChatGPT is a variant of GPT, and related, I wrote a previous article about GPT-3 that can be found here.
Today, I’ll be performing the role of interviewer and you (ChatGPT) will be the interviewee. The interview will be in the form of conversational Q&A and the topic is ChatGPT. I’ll ask questions and you’ll provide answers to the best of your abilities. How does that sound?
That sounds good, I am ready to answer any questions you have about ChatGPT.
Welcome, looking forward to chatting. How was your commute today?
As a machine learning model, I do not have physical presence and thus, I do not have to commute. I am always ready to respond to your questions.
That was a bit of humor and was honestly curious to hear your response. Could you talk about the precursor to ChatGPT?
ChatGPT is a variant of the GPT (Generative Pre-trained Transformer) model, which was first introduced by OpenAI in 2018. GPT was trained on a massive amount of text data and fine-tuned to generate human-like text. The original GPT model was trained on a dataset of about 40 GB of text data, while GPT-2, which was released in 2019, was trained on a much larger dataset of about 570 GB of text data. ChatGPT is a fine-tuned version of GPT-2 specifically designed for conversational modeling.
Could you tell me more about ChatGPT in general, it’s capabilities, features and limitations?
ChatGPT is a state-of-the-art language model developed by OpenAI that is specifically designed for conversational applications. It is based on the GPT-2 architecture and has been fine-tuned on a large dataset of conversational data.
The model is capable of understanding context and generating human-like responses in a conversation. It can be used for various tasks such as question answering, chatbot development, and language translation.
ChatGPT’s capabilities include:
- Generating human-like text in a conversational context
- Answering questions based on the context of the conversation
- Generating coherent and consistent responses in a multi-turn conversation
- Handling a wide range of topics and domains
- Supporting various languages
ChatGPT’s features include:
- Pre-training on a massive amount of conversational data
- Fine-tuning on a smaller dataset for specific tasks
- Large capacity to handle a wide range of conversational tasks
- Generating text with high coherence and consistency
- Handling out-of-vocabulary words and unknown entities.
ChatGPT has some limitations as well:
- Like other language models, it can be prone to perpetuate biases present in the training data.
- It’s also important to note that the model’s performance may depend on the quality and diversity of the fine-tuning dataset, and it’s possible to fine-tune the model on a specific domain or topic to improve its performance.
Could you talk a bit more about the conversational dataset?
Sure, the conversational dataset used to fine-tune ChatGPT is a collection of text data that has been gathered from various sources such as books, websites, and conversational logs. This dataset is used to train the model to understand the context and generate human-like responses in a conversation. The dataset includes various types of conversational data such as dialogues, question-answer pairs, and chat logs.
The size of the dataset used to fine-tune ChatGPT is typically much smaller compared to the dataset used to pre-train the original GPT-2 model, which was about 570 GB. The fine-tuning process allows the model to adapt to the specific task and domain of the conversational data.
The conversational dataset used to fine-tune ChatGPT is diverse, including a wide range of topics and domains, such as customer service, technical support, and general-purpose chatbots. It also supports multiple languages, so the model can be fine-tuned for different languages.
It’s worth noting that the conversational data used to fine-tune the model may come with biases, and it’s important to be aware of and mitigate them, so the model generates fair and unbiased responses.
Could you talk about the fine-tuning process?
The fine-tuning process is a method of adapting a pre-trained language model to a specific task or domain by training it on a smaller dataset. In the case of ChatGPT, the model is fine-tuned on a conversational dataset to adapt it to the task of generating human-like responses in a conversation.
During the fine-tuning process, the model’s parameters are updated to minimize the loss function on the fine-tuning dataset. The fine-tuning dataset is typically much smaller than the pre-training dataset, and the model is trained for a smaller number of iterations.
The fine-tuning process can be done using a variety of techniques, such as transfer learning, where the model is trained on the fine-tuning dataset while keeping some of the pre-trained parameters frozen. Or by using a technique called “Discriminative fine-tuning” where the model is fine-tuned by keeping some of the layers frozen, and only training the last layers.
It’s worth noting that fine-tuning a pre-trained model on a specific task or domain can improve the performance of the model on that task or domain, but it’s important to use a diverse and representative dataset to avoid any biases in the model’s predictions.
Also, fine-tuning a pre-trained model typically requires much less computational resources than training a model from scratch, which makes it possible to use them for various tasks and domains.
Could you list out the domains and topics ChatGPT currently supports?
ChatGPT can support a wide range of domains and topics as it was fine-tuned on a diverse conversational dataset. Some examples of the domains and topics that ChatGPT can support include:
- Customer service: Responding to inquiries and providing assistance to customers.
- Technical support: Answering technical questions and providing solutions to problems.
- General-purpose chatbots: Having general conversations on a wide range of topics.
- Language Translation: Translating sentences from one language to another
- Q&A: Answering questions based on the context of the conversation
- Summarization: Generating summaries of given text.
It’s worth noting that the performance of ChatGPT on specific domains and topics may vary depending on the quality and diversity of the fine-tuning dataset, and it’s possible to fine-tune the model on a specific domain or topic to improve its performance.
Also, as ChatGPT is pre-trained on a diverse dataset, it can handle out-of-vocabulary words and unknown entities, which makes it useful for various tasks and domains.
Can you talk about how out-of-vocabulary words and unknown entities are trained? Maybe give an example?
Out-of-vocabulary (OOV) words and unknown entities are words or phrases that are not present in the vocabulary of the pre-trained language model.
During the pre-training process, ChatGPT learns to recognize and generate words that are similar to the ones in the training dataset. This allows the model to generate coherent and consistent text even when it encounters OOV words or unknown entities that it has not seen during pre-training.
For example, if the model encounters a proper noun that it has not seen during pre-training, such as a name of a person or a place, it can generate text that is coherent and consistent with the context of the conversation.
Additionally, the model can also use context information to generate plausible text for OOV words or unknown entities. For example, if the model encounters a word that it has not seen before, it can use the context of the sentence or the conversation to generate a text that is similar to the one it has seen during pre-training.
It’s worth noting that the model’s ability to handle OOV words and unknown entities may vary depending on the quality and diversity of the pre-training dataset and the context of the conversation.
How many languages do you support? Do some have better support than others?
ChatGPT is a multi-language model that can support a wide range of languages. The model was pre-trained on a diverse dataset that includes text data in various languages such as English, Spanish, French, German, Italian, Portuguese, and Chinese among others.
The performance of the model on specific languages may vary depending on the quality and diversity of the pre-training dataset for that language. Generally, the model has better support for languages that have larger pre-training datasets and more diverse conversational data. For example, the model’s performance on English is generally better than other languages due to the large amount of text data available in English.
To fine-tune the model for a specific language, it is necessary to use a fine-tuning dataset that is representative of the language and the task at hand. This can improve the model’s performance for that language.
It’s worth noting that the model’s ability to understand and generate text in multiple languages can be very helpful in tasks such as language translation and multilingual chatbot development.
Could you talk a bit about support for code generation?
Yes, code generation is one of the capabilities of ChatGPT. The model can generate code in various programming languages, such as Python, JavaScript, and Java.
Code generation with ChatGPT works by providing the model with a prompt that describes the code you want it to generate, along with the necessary context and information about the code. For example, you can provide the model with the prompt “Write a Python function that takes a list and returns the sum of its elements.” and the model will generate the corresponding code.
The model can also generate code for various tasks such as:
- Syntax correction and code completion
- Generating code for specific programming tasks and problems
- Generating code snippets for specific frameworks and libraries
It’s worth noting that the model’s performance in code generation will depend on the quality and diversity of the fine-tuning dataset, and it’s possible to fine-tune the model on a specific domain or task to improve its performance.
It’s also important to note that while the model can generate code that is syntactically correct and runs without error, the generated code may not always be the most efficient or elegant way to solve a problem, and it’s still important to review the code generated by the model and make any necessary adjustments.
As a language model, I can generate code snippets for you with the information that you give me, but I cannot execute the code, that would require an execution environment.
Overall, ChatGPT’s code generation capabilities can be a powerful tool for developers, allowing them to quickly generate code snippets and solve specific programming tasks, but it’s still important to review and understand the generated code before using it in production.
For ChatGPT human input, what is the best way to structure a question? Could you share an example?
When asking a question to ChatGPT, it is important to provide enough context and structure the question in a way that makes it clear and easy for the model to understand. Here are some tips for structuring a question:
- Be specific: Ask a specific question rather than a general one. For example, instead of asking “What is the capital of France?” you can ask “What is the name of the capital city of France?”
- Provide context: Provide enough context to help the model understand the question and generate a relevant response.
- Use proper grammar: Use proper grammar and punctuation to make the question clear and easy to understand.
- Be concise: Keep the question as short and simple as possible.
Here’s an example of a well-structured question:
“What is the GDP per capita of Japan in 2020?”
This question is specific, provides enough context, uses proper grammar and punctuation, and is concise.
It’s worth noting that the model’s ability to understand and answer a question may also depend on the quality and diversity of the fine-tuning dataset, and the model’s performance on specific questions may vary.
Thanks for answering my questions today. So what’s next for ChatGPT, any exciting new features or capabilities upcoming on the roadmap?
I’m glad I could help. As a language model, I don’t have the capability to know about the specific plans or roadmaps of OpenAI, but it’s worth noting that OpenAI is a research organization that is always working on improving their models and developing new capabilities.
The field of natural language processing and machine learning is rapidly evolving, and new techniques and models are being developed all the time. So it’s likely that OpenAI will continue to work on improving ChatGPT and developing new features and capabilities.
In the past, OpenAI has also released new versions of the GPT models, such as GPT-3, which has even more capabilities and features than GPT-2. So it’s possible that OpenAI may release a new version of ChatGPT with improved performance and new capabilities in the future.
It’s worth keeping an eye on OpenAI’s website and blog for updates on new developments and improvements to ChatGPT, and also, other models and capabilities they are working on.
Conclusion
As you can see from this introduction to ChatGPT in its own words, it offers a powerful set of capabilities and features out-of-the-box, and able to speak in natural, sophisticated responses. For this exercise, there were some visible limitations, sometimes not yielding enough details or having the requested information available. Overall, ChatGPT is quite impressive especially given it’s an initial release, and we’ll see what the future brings.
Level Up Coding
Thanks for being a part of our community! Before you go:
- 👏 Clap for the story and follow the author 👉
- 📰 View more content in the Level Up Coding publication
- 🔔 Follow us: Twitter | LinkedIn | Newsletter
🚀👉 Join the Level Up talent collective and find an amazing job
An Interview with ChatGPT was originally published in Level Up Coding on Medium, where people are continuing the conversation by highlighting and responding to this story.
This content originally appeared on Level Up Coding - Medium and was authored by Julian Sitkevich
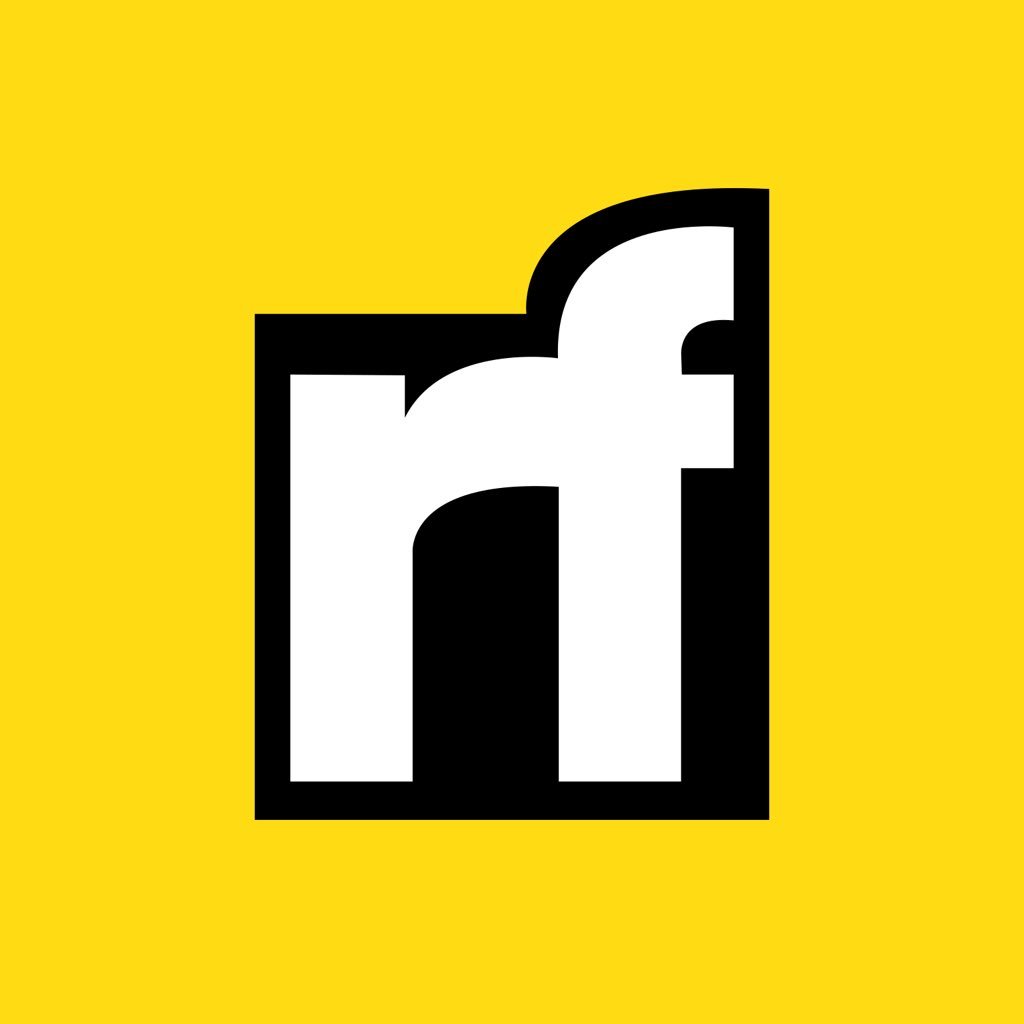
Julian Sitkevich | Sciencx (2023-01-29T11:48:01+00:00) An Interview with ChatGPT. Retrieved from https://www.scien.cx/2023/01/29/an-interview-with-chatgpt/
Please log in to upload a file.
There are no updates yet.
Click the Upload button above to add an update.