This content originally appeared on DEV Community and was authored by Shuveb Hussain
Tables in PDFs are very prevalent and for those of us who are intent on extracting information from PDFs, they often contain very useful information in a very dense format. Processing tables in PDFs, if not the top 10 priority for humanity, it certainly is an important one. Once data from PDFs is extracted, it’s relatively easy to process it using Large Language Models or LLMs. We need to do this in two phases: extract the raw data from tables in PDFs and then pass it to an LLM so that we can extract the data we need as structured JSON. Once we have JSON, it becomes super easy to process in almost any language.
LLMWhisperer for PDF raw text extraction
In this article, we’ll see how to use Unstract’s LLMWhisperer as the text extraction service for PDFs. As you’ll see in some examples we discuss, LLMWhisperer allows us to extract data from PDFs by page, so we can extract exactly what we need. The key difference between various OCR systems and LLMWhisperer is that it outputs data in a manner that is easy for LLMs to process. Also, we don’t have to worry about whether the PDF is a native text PDF, or is made up of scanned images. LLMWhisperer can automatically switch between text and OCR mode as required by the input document.
Before you run the project
The source code for the project can be found here on Github. To successfully run the extraction script, you’ll need 2 API keys. One for LLMWhisperer and the other for OpenAI APIs. Please be sure to read the Github project’s README to fully understand OS and other dependency requirements. You can sign up for LLMWhisperer, get your API key, and process up to 100 pages per day free of charge.
The input documents
Let’s take a look at the documents and the exact pages within the documents from which we need to extract the tables in question. First off, we have a credit card statement from which we’ll need to extract the table of spends, which is on page 2 as you can see in the screenshot below.
The other document we’ll use is one of Apple’s quarterly financial statements, also known as a 10-Q report. From this, we’ll extract Apple’s sales data by different regions in the world. We will extract a table that contains this information from page 14, a screenshot of which is provided below. You can find the sample 10-Q report in the companion Github repository.
The code
Using LLMWhisperer’s Python client, we can extract data from these documents as needed. LLMWhisperer is a cloud service and requires an API key, which you can get for free. LLMWhisperer’s free plan allows you to extract up to 100 pages of data per day, which is more than we need for this example.
def extract_text_from_pdf(file_path, pages_list=None):
llmw = LLMWhispererClient()
try:
result = llmw.whisper(file_path=file_path,
pages_to_extract=pages_list)
extracted_text = result["extracted_text"]
return extracted_text
except LLMWhispererClientException as e:
error_exit(e)
Just calling the whisper()\
method on the client, we’re able to extract raw text from images, native text PDFs, scanned PDFs, smartphone photos of documents, etc.
Here’s the extracted data from page 2 of our credit card statement, which contains the list of spends.
This is the extracted text from page 14 of Apple’s 10-Q document, which contains the sales by geography table towards the end:
Langchain+Pydantic to structure extracted raw tables
Langchain is a popular LLM programming library and Pydantic is one of the parsers it supports for structured data output. If you process a lot of documents of the same type, you might be better off using an open source platform like Unstract which allows you to more visually and interactively develop generic prompts. But for one-off tasks like the example we’re working on, where the main goal is to demonstrate extraction and structuring, Langchain should do just fine. For a comparison of approaches in structuring unstructured documents, read this article: Comparing approaches for using LLMs for structured data extraction from PDFs.
Defining the schema
To use Pydantic with Langchain, we define the schema or structure of the data we want to extract from the unstructured source as Pydantic classes. For the credit card statement spend items, this is how it looks like:
class CreditCardSpend(BaseModel):
spend_date: datetime = Field(description="Date of purchase")
merchant_name: str = Field(description="Name of the merchant")
amount_spent: float = Field(description="Amount spent")
class CreditCardSpendItems(BaseModel):
spend_items: list[CreditCardSpend] = Field(description="List of spend items from the credit card statement")
Looking at the definitions, CreditCardSpendItems\
is just a list of CreditCardSpend\
, which defines the line item schema, which contains the spend date, merchant name and amount spent.
For the 10-Q regional sales details, the schema looks like the following:
class RegionalFinancialStatement(BaseModel):
quarter_ending: datetime = Field(description="Quarter ending date")
net_sales: float = Field(description="Net sales")
operating_income: float = Field(description="Operating income")
ending_type: str = Field(description="Type of ending. Set to either '6-month' or '3-month'")
class GeographicFinancialStatement(BaseModel):
americas: list[RegionalFinancialStatement] = Field(description="Financial statement for the Americas region, "
"sorted chronologically")
europe: list[RegionalFinancialStatement] = Field(description="Financial statement for the Europe region, sorted "
"chronologically")
greater_china: list[RegionalFinancialStatement] = Field(description="Financial statement for the Greater China "
"region, sorted chronologically")
japan: list[RegionalFinancialStatement] = Field(description="Financial statement for the Japan region, sorted "
"chronologically")
rest_of_asia_pacific: list[RegionalFinancialStatement] = Field(description="Financial statement for the Rest of "
"Asia Pacific region, sorted "
"chronologically")
Constructing the prompt and calling the LLM
The following code uses Langchain to let us define the prompts to structure data from the raw text like we need it. The compile_template_and_get_llm_response()\
function has all the logic to compile our final prompt from the preamble, the instructions from the Pydantic class definitions along with the extracted raw text. It then calls the LLM and returns the JSON response.
def compile_template_and_get_llm_response(preamble, extracted_text, pydantic_object):
postamble = "Do not include any explanation in the reply. Only include the extracted information in the reply."
system_template = "{preamble}"
system_message_prompt = SystemMessagePromptTemplate.from_template(system_template)
human_template = "{format_instructions}\n\n{extracted_text}\n\n{postamble}"
human_message_prompt = HumanMessagePromptTemplate.from_template(human_template)
parser = PydanticOutputParser(pydantic_object=pydantic_object)
chat_prompt = ChatPromptTemplate.from_messages([system_message_prompt, human_message_prompt])
request = chat_prompt.format_prompt(preamble=preamble,
format_instructions=parser.get_format_instructions(),
extracted_text=extracted_text,
postamble=postamble).to_messages()
chat = ChatOpenAI()
response = chat(request, temperature=0.0)
print(f"Response from LLM:\n{response.content}")
return response.content
def extract_cc_spend_from_text(extracted_text):
preamble = ("You're seeing the list of spend items from a credit card statement and your job is to accurately "
"extract the spend date, merchant name and amount spent for each transaction.")
return compile_template_and_get_llm_response(preamble, extracted_text, CreditCardSpendItems)
def extract_financial_statement_from_text(extracted_text):
preamble = ("You're seeing the financial statement for a company and your job is to accurately extract the "
"revenue, cost of revenue, gross profit, operating income, net income and earnings per share.")
return compile_template_and_get_llm_response(preamble, extracted_text, GeographicFinancialStatement)
The output: PDF to JSON
Let’s examine the JSON output from the credit card statement table and the region-wise sales table. Here’s the structured JSON from the credit card spend items table first:
{
"spend_items": [
{
"spend_date": "2024-01-04",
"merchant_name": "LARRY HOPKINS HONDA 7074304151 CA",
"amount_spent": 265.40
},
{
"spend_date": "2024-01-04",
"merchant_name": "CICEROS PIZZA SAN JOSE CA",
"amount_spent": 28.18
},
{
"spend_date": "2024-01-05",
"merchant_name": "USPS PO 0545640143 LOS ALTOS CA",
"amount_spent": 15.60
},
{
"spend_date": "2024-01-07",
"merchant_name": "TRINETHRA SUPER MARKET CUPERTINO CA",
"amount_spent": 7.92
},
{
"spend_date": "2024-01-04",
"merchant_name": "SPEEDWAY 5447 LOS ALTOS HIL CA",
"amount_spent": 31.94
},
{
"spend_date": "2024-01-06",
"merchant_name": "ATT*BILL PAYMENT 800-288-2020 TX",
"amount_spent": 300.29
},
{
"spend_date": "2024-01-07",
"merchant_name": "AMZN Mktp US*RT4G124P0 Amzn.com/bill WA",
"amount_spent": 6.53
},
{
"spend_date": "2024-01-07",
"merchant_name": "AMZN Mktp US*RT0Y474Q0 Amzn.com/bill WA",
"amount_spent": 21.81
},
{
"spend_date": "2024-01-05",
"merchant_name": "HALAL MEATS SAN JOSE CA",
"amount_spent": 24.33
},
[some items removed for concision]
]
}
Excellent! We got exactly what we were looking for. Next, let’s look at the JSON output structured from the regional sales table from Apple’s 10-Q PDF.
{
"americas": [
{
"quarter_ending": "2024-03-30T00:00:00Z",
"net_sales": 37273,
"operating_income": 15074,
"ending_type": "3-month"
},
{
"quarter_ending": "2023-04-01T00:00:00Z",
"net_sales": 37784,
"operating_income": 13927,
"ending_type": "3-month"
},
{
"quarter_ending": "2024-03-30T00:00:00Z",
"net_sales": 87703,
"operating_income": 35431,
"ending_type": "6-month"
},
{
"quarter_ending": "2023-04-01T00:00:00Z",
"net_sales": 87062,
"operating_income": 31791,
"ending_type": "6-month"
}
],
"europe": [
{
"quarter_ending": "2024-03-30T00:00:00Z",
"net_sales": 24123,
"operating_income": 9991,
"ending_type": "3-month"
},
{
"quarter_ending": "2023-04-01T00:00:00Z",
"net_sales": 23945,
"operating_income": 9368,
"ending_type": "3-month"
},
{
"quarter_ending": "2024-03-30T00:00:00Z",
"net_sales": 54520,
"operating_income": 22702,
"ending_type": "6-month"
},
{
"quarter_ending": "2023-04-01T00:00:00Z",
"net_sales": 51626,
"operating_income": 19385,
"ending_type": "6-month"
}
],
"greater_china": [
{
"quarter_ending": "2024-03-30T00:00:00Z",
"net_sales": 16372,
"operating_income": 6700,
"ending_type": "3-month"
},
{
"quarter_ending": "2023-04-01T00:00:00Z",
"net_sales": 17812,
"operating_income": 7531,
"ending_type": "3-month"
},
{
"quarter_ending": "2024-03-30T00:00:00Z",
"net_sales": 37191,
"operating_income": 15322,
"ending_type": "6-month"
},
{
"quarter_ending": "2023-04-01T00:00:00Z",
"net_sales": 41717,
"operating_income": 17968,
"ending_type": "6-month"
}
],
"japan": [
"Some items removed for concision"
],
"rest_of_asia_pacific": [
"Some items removed for concision"
]
}
This output is as we expected, too. With this kind of structured output, it becomes very easy for us to process complex information in these kinds of tables further. The key to easy extraction of structured information is the availability of cleanly formatted input tables and LLMWhisperer here plays a key role in that extraction even for scanned documents or documents that are just smartphone photos.
Links to libraries, packages, and code
- LLMWhisperer: A general purpose text extraction service that extracts data from images and PDFs, preparing it and optimizing it for consumption by Large Language Models or LLMs.
- LLMwhisperer Python client on PyPI | Try LLMWhisperer Playground for free | Learn more about LLMWhisperer
- The code for this guide can be found in this GitHub Repository
- Pydantic: Use Pydantic to declare your data model. This output parser allows users to specify an arbitrary Pydantic Model and query LLMs for outputs that conform to that schema.
For the curious. Who am I and why am I writing about PDF text extraction?
I'm Shuveb, one of the co-founders of Unstract.
Unstract is a no-code platform to eliminate manual processes involving unstructured data using the power of LLMs. The entire process discussed above can be set up without writing a single line of code. And that’s only the beginning. The extraction you set up can be deployed in one click as an API or ETL pipeline.
With API deployments you can expose an API to which you send a PDF or an image and get back structured data in JSON format. Or with an ETL deployment, you can just put files into a Google Drive, Amazon S3 bucket or choose from a variety of sources and the platform will run extractions and store the extracted data into a database or a warehouse like Snowflake automatically. Unstract is an Open Source software and is available at https://github.com/Zipstack/unstract.
If you want to quickly try it out, signup for our free trial. More information here.
Note: I originally posted this on the Unstract blog a couple of weeks ago.
This content originally appeared on DEV Community and was authored by Shuveb Hussain
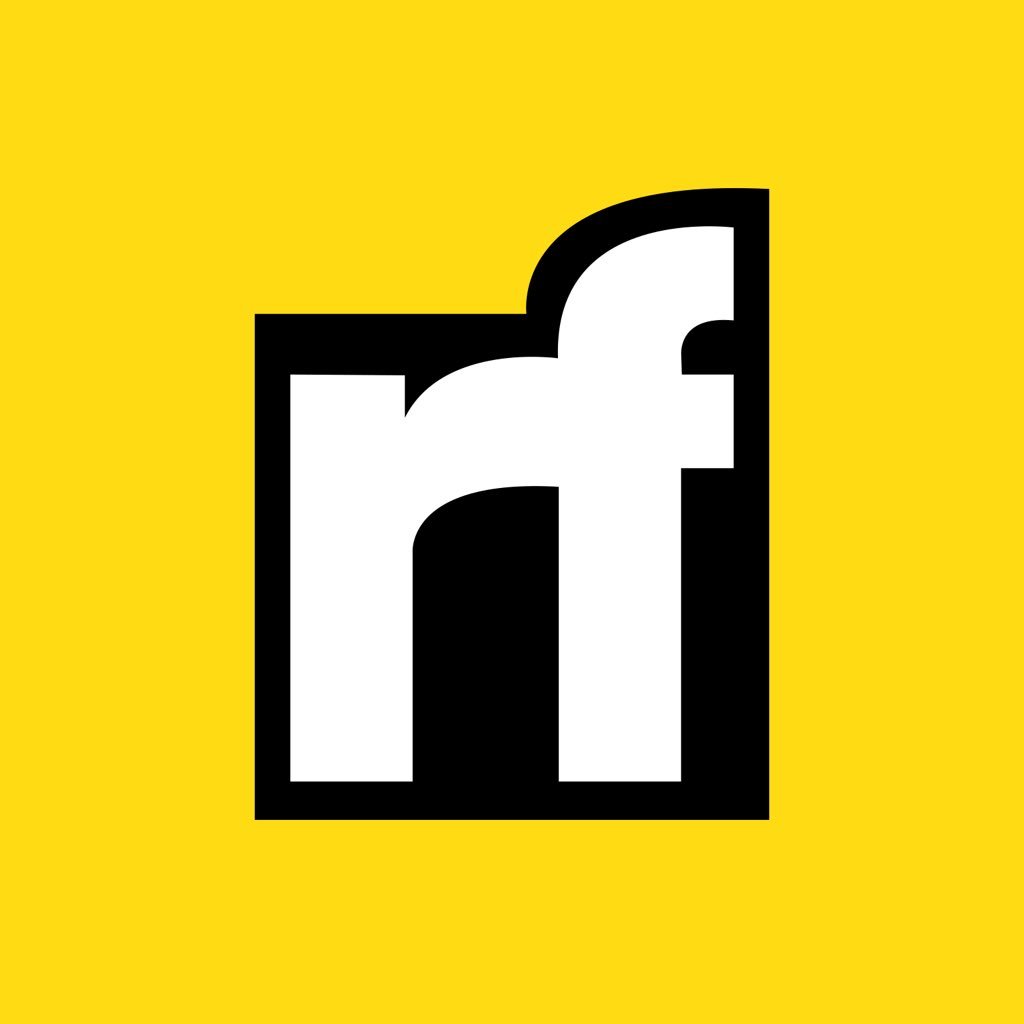
Shuveb Hussain | Sciencx (2024-07-11T14:44:20+00:00) Table Extraction and Processing from PDFs – A Tutorial. Retrieved from https://www.scien.cx/2024/07/11/table-extraction-and-processing-from-pdfs-a-tutorial/
Please log in to upload a file.
There are no updates yet.
Click the Upload button above to add an update.