This content originally appeared on HackerNoon and was authored by David Stephen
If an individual were temporarily indisposed, it is possible to assume that most of the memory the individual has might be intact, but the relays to make the memories available may have plummeted.
\ This highlights that memory is not only about the stored information but also about the relays that make it accessible. It is possible to define human memory, not just by types, but by specifications of relays for availability, such that those for speech at some point may be different from those from imagination, or those for learning from those for doing. Simply, outputs from human memory are sometimes a result of reach or relays, not just stored info.
\ Relays can also be used to explain how Large language models [LLMs] work. LLMs are trained on vast amounts of internet data. This training can be assumed to be relays. Prompts to, and outputs from, LLMs can also be assumed to be relays, across data. Simply, LLMs work because they can relay predictively, with correct matches for several use cases.
\ Though these relays still have their weakness—like confabulations, hallucinations, slops in generated videos and images, and so forth—the better the relays or math functions that are developed for digital memory, the closer they might come to human intelligence.
\ Artificial general intelligence [AGI] or artificial superintelligence [ASI] could get closer with improved relays across digital memory, in parallel to relays that result in intelligence in the human mind.
\ AGI can be described as relay equivalents to those in the human mind, that define human intelligence. Relays may also make and specify extensions like—levels of control and base subjectivity.
\ Though the internet already has more information than any individual’s brain, AGI may be as good as relays that use the information, for similarities to human intelligence, not just the presence of information. This is similar to the human mind, where presence [of memory] does not mean available [in an instance].
\ The human mind is theorized to have functions and features. Though functions are shaped by relays, features are dominated by relays. Functions can be categorized into memory, feeling, emotion, and modulation of internal senses. Functions have several subcategories including—intelligence, planning, reasoning, pain, delight, cold, heat, and so forth. Functional instances are determined by how they are graded or qualified by features. Features are types of relays that bring those functions to use, including their intensities. The human mind is theorized to be the collection of all the electrical and chemical signals with their interactions and features, in sets, in clusters of neurons, across the central and peripheral nervous systems.
\ Features [or relays] include pre-/prioritization, old and new sequences, early splits, principal spot, thick/thin sets, volume changes, diameter constant spaces, and others. These relays are postulated to directly grade what is learned and experienced.
\ AGI would not be a measure of outcomes, but a measure of relays, since outcomes may be great yet deficient. For example, LLMs are excellent at predictions, but lacking in correction and in the knowledge of consequences. They are cool at several types of pattern-matching but seem to be unidirectional in several outputs, which is not the case for the human mind.
\
Two of several questions about ASI are—can it have control and can it have subjectivity? In the human mind, control, free will or intent can be defined as functions that can be intentionally adjusted, like chewing, sitting, turning the neck and so forth. There are functions that cannot be controlled like the spleen and so forth, at least directly like speaking, waving and so on. Subjectivity is attachment, personalization or self-association, away from detachment, depersonalization or dissociation.
\ Control [or intent] and subjectivity [or self] are postulated to be mechanized in the same [sets of electrical and chemical] signals for functions. While control and subjectivity may not be described as standalone functions or information—such that something must often be controlled and there has to be an experience, for the self to present—they are also subcategories, where relays get to and determine their intensities.
\ For AGI, it could be possible that rows within high-dimensional vectors become aspects of control, and some columns—aspects of subjectivity. Control and subjectivity would be embeddings that can be used alone and have to be relayed to, for availability, unlike for humans. Simply, AGI would use vectors to control vectors and use vectors to [be subjective or] have subjectivity, conceptually.
\ Conceptual brain science could be useful in grading LLMs to AGI, becoming another direction for computational and theoretical neuroscience to shape progress in AI.
\ There is a recent editorial in Nature, The New NeuroAI, stating that, “Overall, the extent of the role of neuroscience in AI research, and that of AI in neuroscience research, remain open questions for the future. However, these two fields are deeply linked, and the exchange of ideas between them continues to evolve. The pursuit of more powerful artificial neural systems in leading AI research labs, particularly those affiliated with tech companies, is currently focused on engineering. This pursuit emphasizes further scaling up of complex architectures such as transformers, rather than integrating insights from neuroscience.”
\ There is a recent paper in Nature, Reservoir-computing based associative memory and itinerancy for complex dynamical attractors, stating that “A neural network capable of memorizing and retrieving multiple memory states is also a multifunctional neural network. When different states are recalled, the neural network exhibits distinct dynamical behaviors. The idea of multifunctional RNNs has been discussed previously with different forms of implementation. In particular, in the index-based approach, an index parameter is introduced to modulate the functionality of the neural networks and store different states with different index values, but recalling the memorized states was a challenge, for which control may be necessary.”
\ There is a new podcast on The Robot Report, New AI, controls make robots easier to program and deploy, discussing a “new industrial robotics robot control architecture.”
\ In conclusion, the concept of relays in both human memory and artificial intelligence (AI) highlights the crucial role of accessibility mechanisms in achieving functionality. As such, the pursuit of artificial general intelligence (AGI) and artificial superintelligence (ASI) may hinge on developing sophisticated relay systems that better mirror the intricate functions of the human mind.
\ \ \
This content originally appeared on HackerNoon and was authored by David Stephen
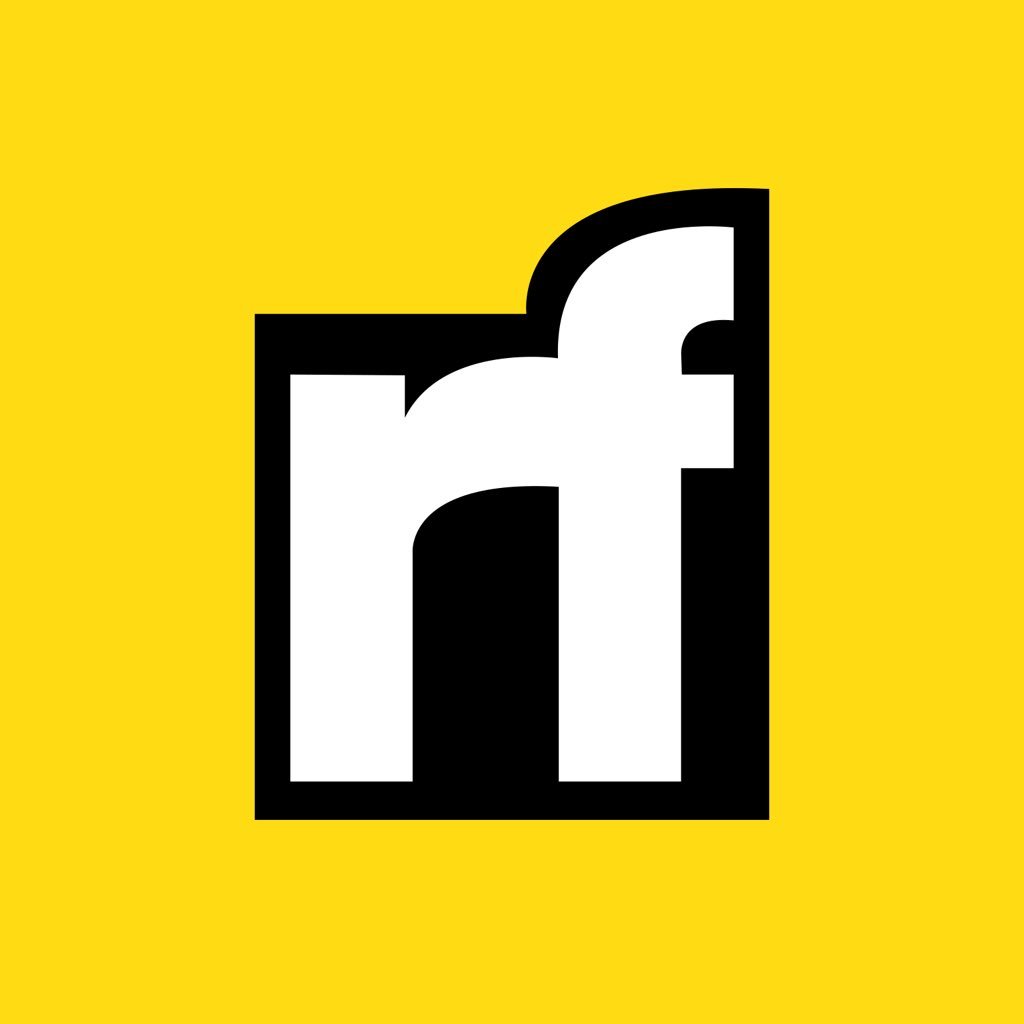
David Stephen | Sciencx (2024-07-31T22:14:10+00:00) Brain Science: Benchmarks for AGI, LLMs—Superintelligence. Retrieved from https://www.scien.cx/2024/07/31/brain-science-benchmarks-for-agi-llms-superintelligence/
Please log in to upload a file.
There are no updates yet.
Click the Upload button above to add an update.