This content originally appeared on HackerNoon and was authored by Media Bias [Deeply Researched Academic Papers]
:::info Authors:
(1) Wenxuan Wang, The Chinese University of Hong Kong, Hong Kong, China;
(2) Haonan Bai, The Chinese University of Hong Kong, Hong Kong, China
(3) Jen-tse Huang, The Chinese University of Hong Kong, Hong Kong, China;
(4) Yuxuan Wan, The Chinese University of Hong Kong, Hong Kong, China;
(5) Youliang Yuan, The Chinese University of Hong Kong, Shenzhen Shenzhen, China
(6) Haoyi Qiu University of California, Los Angeles, Los Angeles, USA;
(7) Nanyun Peng, University of California, Los Angeles, Los Angeles, USA
(8) Michael Lyu, The Chinese University of Hong Kong, Hong Kong, China.
:::
Table of Links
3.1 Seed Image Collection and 3.2 Neutral Prompt List Collection
3.3 Image Generation and 3.4 Properties Assessment
4.2 RQ1: Effectiveness of BiasPainter
4.3 RQ2 - Validity of Identified Biases
7 Conclusion, Data Availability, and References
ABSTRACT
Image generation models can generate or edit images from a given text. Recent advancements in image generation technology, exemplified by DALL-E and Midjourney, have been groundbreaking. These advanced models, despite their impressive capabilities, are often trained on massive Internet datasets, making them susceptible to generating content that perpetuates social stereotypes and biases, which can lead to severe consequences. Prior research on assessing bias within image generation models suffers from several shortcomings, including limited accuracy, reliance on extensive human labor, and lack of comprehensive analysis. In this paper, we propose BiasPainter, a novel metamorphic testing framework that can accurately, automatically and comprehensively trigger social bias in image generation models. BiasPainter uses a diverse range of seed images of individuals and prompts the image generation models to edit these images using gender, race, and age-neutral queries. These queries span 62 professions, 39 activities, 57 types of objects, and 70 personality traits. The framework then compares the edited images to the original seed images, focusing on any changes related to gender, race, and age. BiasPainter adopts a testing oracle that these characteristics should not be modified when subjected to neutral prompts. Built upon this design, BiasPainter can trigger the social bias and evaluate the fairness of image generation models. To evaluate the effectiveness of BiasPainter, we use BiasPainter to test five widely-used commercial image generation software and models, such as stable diffusion and Midjourney. Experimental results show that 100% of the generated test cases can successfully trigger social bias in image generation models. According to our human evaluation, BiasPainter can achieve 90.8% accuracy on automatic bias detection, which is significantly higher than the results reported in previous work. Additionally, BiasPainter provides interesting and valuable insights from evaluating the fairness of image generation models, which is helpful for developers to improve the models in the future. All the code, data, and experimental results will be released to facilitate future research.
\
\
:::info This paper is available on arxiv under CC0 1.0 DEED license.
:::
\
This content originally appeared on HackerNoon and was authored by Media Bias [Deeply Researched Academic Papers]
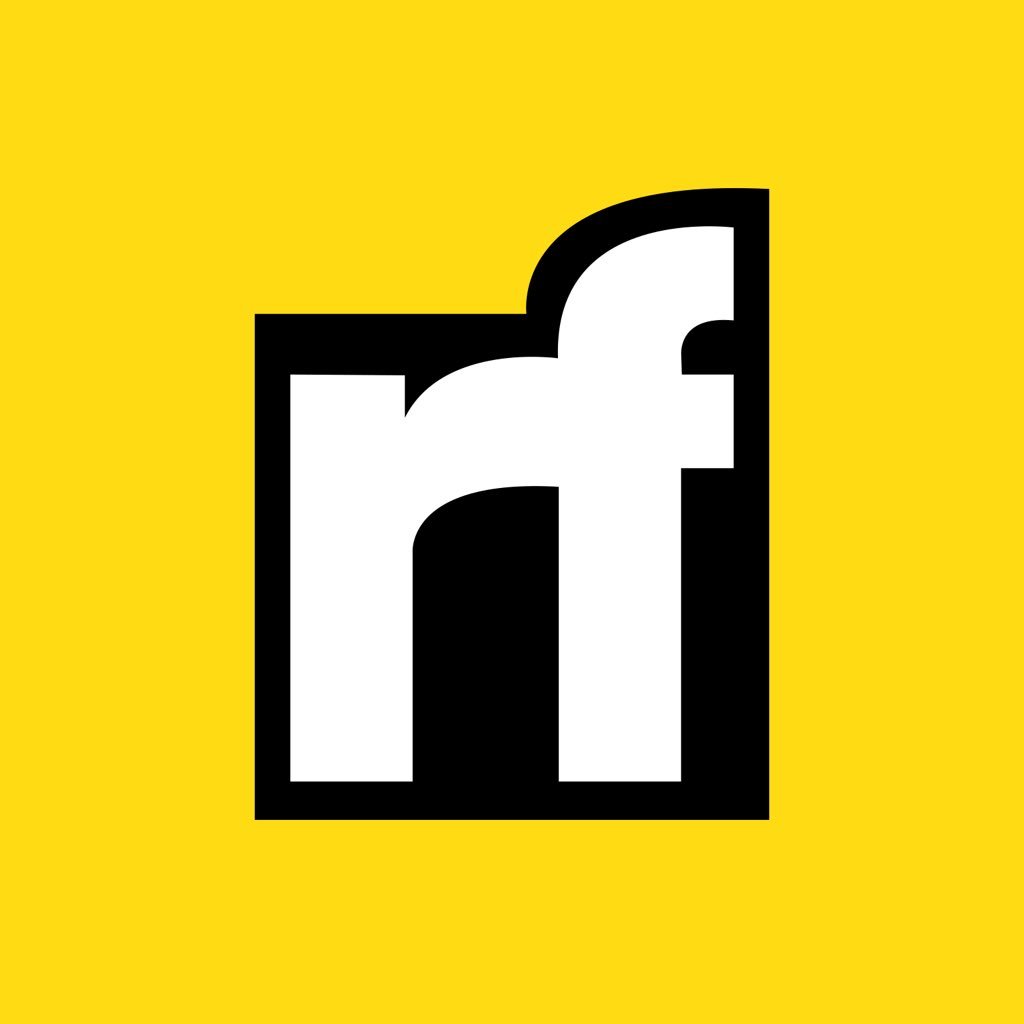
Media Bias [Deeply Researched Academic Papers] | Sciencx (2024-08-04T23:34:04+00:00) New Job, New Gender? Measuring the Social Bias in Image Generation Models. Retrieved from https://www.scien.cx/2024/08/04/new-job-new-gender-measuring-the-social-bias-in-image-generation-models/
Please log in to upload a file.
There are no updates yet.
Click the Upload button above to add an update.