This content originally appeared on HackerNoon and was authored by Writings, Papers and Blogs on Text Models
:::info Authors:
(1) Rafael Rafailo, Stanford University and Equal contribution; more junior authors listed earlier;
(2) Archit Sharma, Stanford University and Equal contribution; more junior authors listed earlier;
(3) Eric Mitchel, Stanford University and Equal contribution; more junior authors listed earlier;
(4) Stefano Ermon, CZ Biohub;
(5) Christopher D. Manning, Stanford University;
(6) Chelsea Finn, Stanford University.
:::
Table of Links
4 Direct Preference Optimization
7 Discussion, Acknowledgements, and References
\ A Mathematical Derivations
A.1 Deriving the Optimum of the KL-Constrained Reward Maximization Objective
A.2 Deriving the DPO Objective Under the Bradley-Terry Model
A.3 Deriving the DPO Objective Under the Plackett-Luce Model
A.4 Deriving the Gradient of the DPO Objective and A.5 Proof of Lemma 1 and 2
\ B DPO Implementation Details and Hyperparameters
\ C Further Details on the Experimental Set-Up and C.1 IMDb Sentiment Experiment and Baseline Details
C.2 GPT-4 prompts for computing summarization and dialogue win rates
\ D Additional Empirical Results
D.1 Performance of Best of N baseline for Various N and D.2 Sample Responses and GPT-4 Judgments
Mathematical Derivations
A.1 Deriving the Optimum of the KL-Constrained Reward Maximization Objective
In this appendix, we will derive Eq. 4. Analogously to Eq. 3, we optimize the following objective:
\
\ where we have partition function:
\
\
\
:::info This paper is available on arxiv under CC BY-NC-ND 4.0 DEED license.
:::
\
This content originally appeared on HackerNoon and was authored by Writings, Papers and Blogs on Text Models
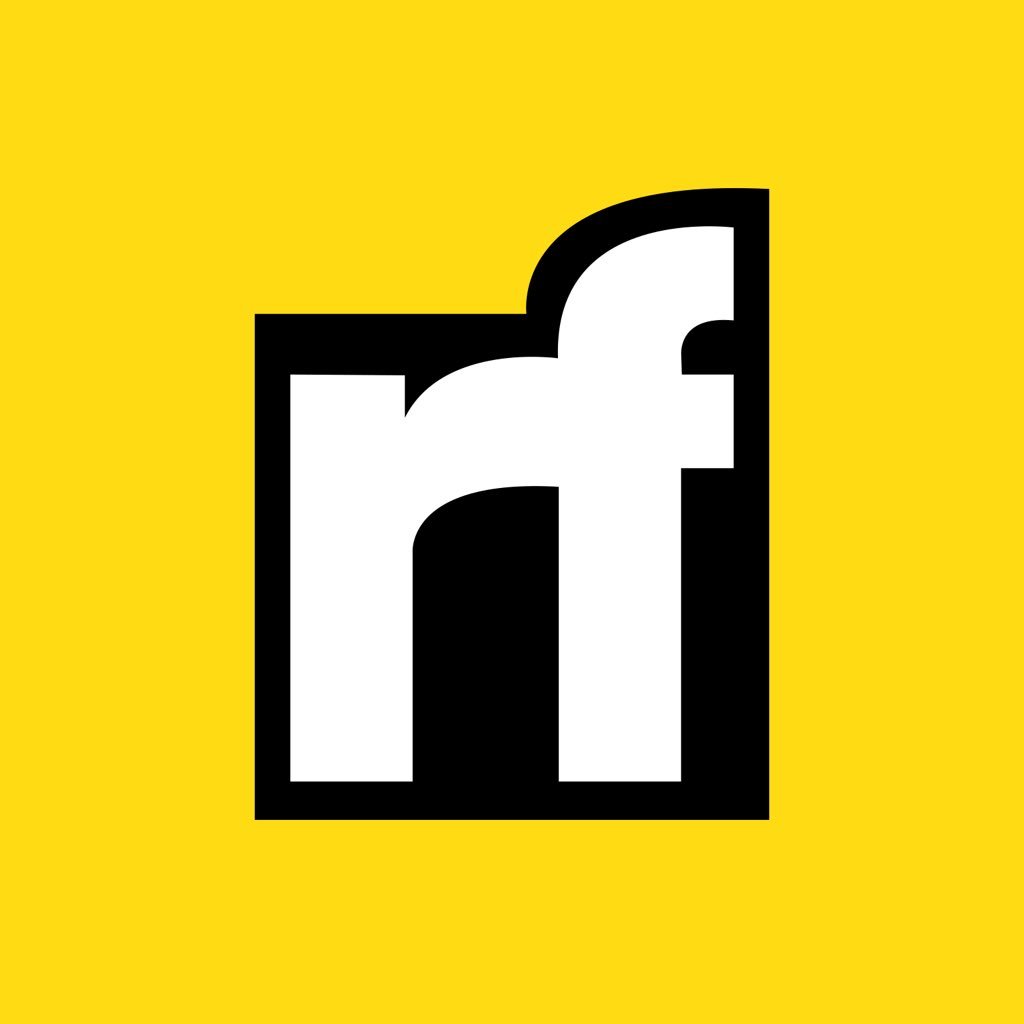
Writings, Papers and Blogs on Text Models | Sciencx (2024-08-25T21:13:38+00:00) Deriving the Optimum of the KL-Constrained Reward Maximization Objective. Retrieved from https://www.scien.cx/2024/08/25/deriving-the-optimum-of-the-kl-constrained-reward-maximization-objective/
Please log in to upload a file.
There are no updates yet.
Click the Upload button above to add an update.