This content originally appeared on DEV Community and was authored by jinesh vora
Table of Contents
- Introduction: Taming the Data Landscape
- Understanding Data Lakes: Big Data Storage Foundations
- The Rise of Data Mesh: The Next Paradigm Shift in Data Management
- Key Differences Between Data Lakes and Data Mesh
- Gains from Adopting a Data Mesh Architecture
- Challenges in Implementing Data Mesh
- Integration of Data Lakes and Data Mesh: The Hybrid Approach
- Use Cases of Real-World Applications of Data Mesh and Data Lakes
- The Future of Data Management and Analytics
- Conclusion: Embracing Change in Data Strategies
Introduction: Navigating the Data Landscape
The ever-changing world of data management and analytics develops new ways for organizations to leverage data. As businesses generate and collect vast volumes of information, traditional ways of data management today are faced with increasing trends like lakes and mesh.
It's an important understanding that will allow data professionals to further optimize their data strategies, driving meaningful insights.
The data lake had been the most popular solution for storing huge volumes of structured and unstructured data for a number of years. However, with organizations growing and their needs getting complex regarding data handling, the limitations of data lakes increase, allowing more modern architectures such as the data mesh to take over. In the rest of the article, we shall take a deep dive into data lakes-what are their characteristics, benefits, and challenges-and the same goes with the data mesh, too.
Data Lakes: Understand the Basics of Big Data Storage
A data lake is a unified repository that stores raw data of any type, whether it be structured, semi-structured, or unstructured, in native format. This makes it easier for businesses to ingest and manage large volumes of data without the pre-requisites of schema definitions. Data lakes are perfectly suited to applications where big data is used, or data is used in machine learning and analytics.
Among the major advantages of data lakes, one would emphasize that these can support a wide array of data types and sources. The firms can store everything from transactional data and log files to social media posts and sensor data, and that ultimately makes analysis and insight derivation from varied data sets much easier. Besides, data lakes often rely on cloud storage solutions, thereby opening wide opportunities for data management in an agile and cost-efficient manner.
Of course, data lakes have their own problems. The absence of structure sometimes creates issues in data governance, quality control, and accessibility. It may be difficult for an organization to maintain integrity over data as its volume grows in a data lake and always ensure that the required information is presented before the users. Because of this, other alternative methods have been explored concerning data management, one of which involves the use of a data mesh.
The Rise of the Data Mesh: A Paradigm Shift to Data Management
Data mesh is the latest, architectural paradigm which has emerged recently and refers to decentralized data management-oriented domains. It introduces a new twist into data management, deviating from the usual data lakes where storage and processing are carried out centralized. This encourages distribution of the ownership of data across various business domains, which in turn, promotes team responsibility for own data and hence collaboration with agility.
The concept of data mesh, first mooted by Zhamak Dehghani, is based on the claim that growing complexity for data ecosystems necessitates an architecture quite unlike monolithic. What it means, in other words, is that instead of maintaining big monolithic blocks of architecture, companies should treat the data as a product and empower domain-specific teams to manage their own pipelines in order for organizations to enhance data accessibility to users for making informed decisions.
Data mesh also fosters self-serve data infrastructure, where teams can utilize, share, and use data all on their own without the help or support of some central data team. It goes a step further than this, though, as this decentralized approach further deepens the extent to which data is democratized while simultaneously smashing bottlenecks, improving an organization's response time to react to the evolution of changing business needs.
Key Differences Between Data Lakes and Data Mesh
Where data lakes and data mesh both enable data management and analytics, the methods and fundamental philosophies differ radically.
Architecture: Data lakes are centralized storage facilities that house all data in one location, while the architecture of data mesh is decentralized, distributing ownership of data across multiple business domains. This basic difference impacts the way data is managed, accessed, and used within an organization.
Ownership of Data: While the centrally located data team normally looks after the data with regards to ingestion, processing, and governance in a data lake, the data mesh pushes this ownership into domain-specific teams, each empowered with data management practices to best suit their specific needs and use cases.
Governance: The most prevalent problem encountered in data lakes pertains to data
This prevents governance at large enterprises where centralized data management brings along a lot of inconsistencies and accessibility. Data mesh, on the other hand, promotes federated governance; this means each domain is responsible for its data against standards and overall compliance requirements across domains.
Scalability: With huge volumes of data, data lakes often grow out of control, making them slow and hard to manage, especially issues related to the quality of the data. Data mesh addresses scalability by letting individual domains build their own optimized pipelines for data and the storage solutions of choice, leading to a more agile and responsive data architecture.
Gaining an understanding of these differences will help organizations in making informed decisions on which data management strategies will work best to meet their needs and support their goals.
Benefits of Adopting a Data Mesh Architecture
Implementing a data mesh architecture offers numerous benefits to powerfully help an organization enhance its capabilities in managing data, including the following:
Increased Agility: A Data mesh architecture gives organizations the ability to be more agile. With data ownership decentralized, organizations can better react to changing business needs. Domain teams are able to make decisions about data management and analytics without having to wait for a centralized data team to review or approve such requests. This helps facilitate speedier insights and thus faster decision-making.
Better Collaboration: Data mesh empowered domain teams that were motivated and encouraged to share data and insights across the organizations. It makes sure the environment of a data-driven culture of decision-making and allows teams to use each other's expertise.
Data Quality Improved: Now that the ownership has fallen on the domain teams, they placed more emphasis on the maintenance of data quality and integrity. In essence, the teams are keen to ensure their data is accurate, up-to-date, and relevant for certain use cases.
Scalability: Data mesh allows organizations to scale more and better regarding data management. New sources of data are going to emerge, and new use cases are likely to be thought of. Thus, the pipelines and storage solutions in domain teams can keep pace with such evolving needs without the bottlenecks of a centralized architecture.
Building upon this data mesh architecture enables an organization to open up new avenues for innovation and growth, preparing them to thrive in an increasingly data-driven world.
Challenges in Deploying Data Mesh
An appeal of the data mesh is strong, but organizations are also reminded of the challenges with its implementation:
Organizational Culture: There is a sea change in the organizational culture needed for implementing a data mesh architecture. There needs to be the adoption of ideas of data ownership and collaboration with other teams. Those changes also come with some mind shift and practice changes. Good leadership with proper communication is required to adapt this organizational change.
Technical Complexity: A mesh architecture for data mainly fosters technical complexity in the arenas of data integration and governance. Organizations would be required to invest in the right set of tools and technologies that support decentralized data management so that data is kept accessible and compliant.
Skill Gaps: Since data mesh advocates ownership that is domain-led, skill gaps in teams are very much going to be there. Hence, domain teams who have adequate skill sets for managing data effectively would make sure that data mesh implementation is a success.
Governance Challenges: While data mesh promotes federated governance, it will lead to inconsistencies if not governed properly. Clear guidelines and conventions would need to be defined by the organizations so that teams work in cohesion but still maintain their autonomous nature.
Organizations address these challenges when they successfully implement data mesh architecture, which brings several benefits of the Data Mesh architecture.
Hybrid Data Lakes and Data Mesh
The organizations would stand a better chance of a hybrid approach, incorporating some of the best qualities of both rather than adopting either a data lake or data mesh. There could be a data lake as a centralized storage for raw data, while a framework of data mesh enables decentralized data management and ownership.
Each brings together scalability and flexibility offered by data lakes with driving better collaboration and agility using the principles of data mesh. This hybrid model will let an organization store huge amounts of data in a cost-effective manner while giving domain teams the power to manage their data and analytics processes.
This will require careful planning because a hybrid approach would consider the very specific needs and objectives of the organization. By aligning data management practices in line with business objectives, the organization will have a robust data-ecosystem to drive innovation, ensured support for data-driven decision-making.
Application of Data Mesh and Data Lakes in Real World
Integration of data lakes and data mesh has transformed many applications across different industries. Following are some notable examples:
E-commerce: Online retail giants store massive amounts of customer data, transaction records, and product information in data lakes. A data mesh architecture gives that data to the marketing or sales team for self-service analytics that can provide personalized recommendations and targeted marketing campaigns.
Healthcare: Data lakes store patient records, clinical data, and research findings in healthcare. Taking a data mesh approach will make it easier for different departments within healthcare organizations to enable ownership of the data in their possession, with adherence to regulations and data quality.
Finance: Banks use data lakes to store transactional data, market data, and customer information. With the data mesh architecture, they are able to empower risk and compliance teams to have the analysis capability themselves, thus better fraud detection by these teams and managing risks more effectively.
Manufacturing will store sensor data, production metrics, and supply chain information in the data lake. The application of a data mesh approach would enable production and operations teams to perform real-time analysis of data for process optimization and downtown reduction.
These two examples show how data lakes and data mesh together create innovation streams that improve business outcomes.
The Future of Data Management and Analytics
The future of the approaches adopted by organizations in dealing with data management and analytics will keep evolving as they face the intricacies of the complexities involved. Data mesh has emerged as a reflection of the increasing recognition of the need for decentralized data management and ownership that is driven by the needs of a domain.
Especially in terms of emerging technologies, the future will literally be shaped by artificial intelligence and machine learning. Integrating data, automating rules and governance processes, building platform-as-a-service capabilities for advanced analytics-new technology can do it all in a way that helps organizations glean deeper insights from their data.
More importantly, the increasing regulation over data privacy will drive the point home that organizations should implement proper data governance and compliance in their data management strategy. A data mesh architecture will enable federated governance while empowering domain teams to manage their data responsibly.
Conclusion: Riding the Change in Data Strategies
This transition from a Data Lake to a Data Mesh could be a gigantic leap for the organizations governing and analyzing data. Clearly, understanding the traits of both architectures, advantages, and disadvantages will help an organization make well-informed decisions regarding its data strategy and harness to full potential the data available.
As data-driven decision-making continues to see an upward surge in demand, so will the preparedness and skill of data professionals to master these concepts.
In this respect, enrollment in the Data Science course in Thane at the Thane center provides the learner with exposures and hands-on experience in data management practices that will surely help them to stand out in this emerging scenario.
The reasons that make organizations move towards data strategy adoption are, in turn, setting them up to lead into the future: data-driven, an organization will unlock its data's power and drive innovation across operations. From a data lake, a data mesh, or a hybrid, the journey of effective management of data will provide for business transformations.
This content originally appeared on DEV Community and was authored by jinesh vora
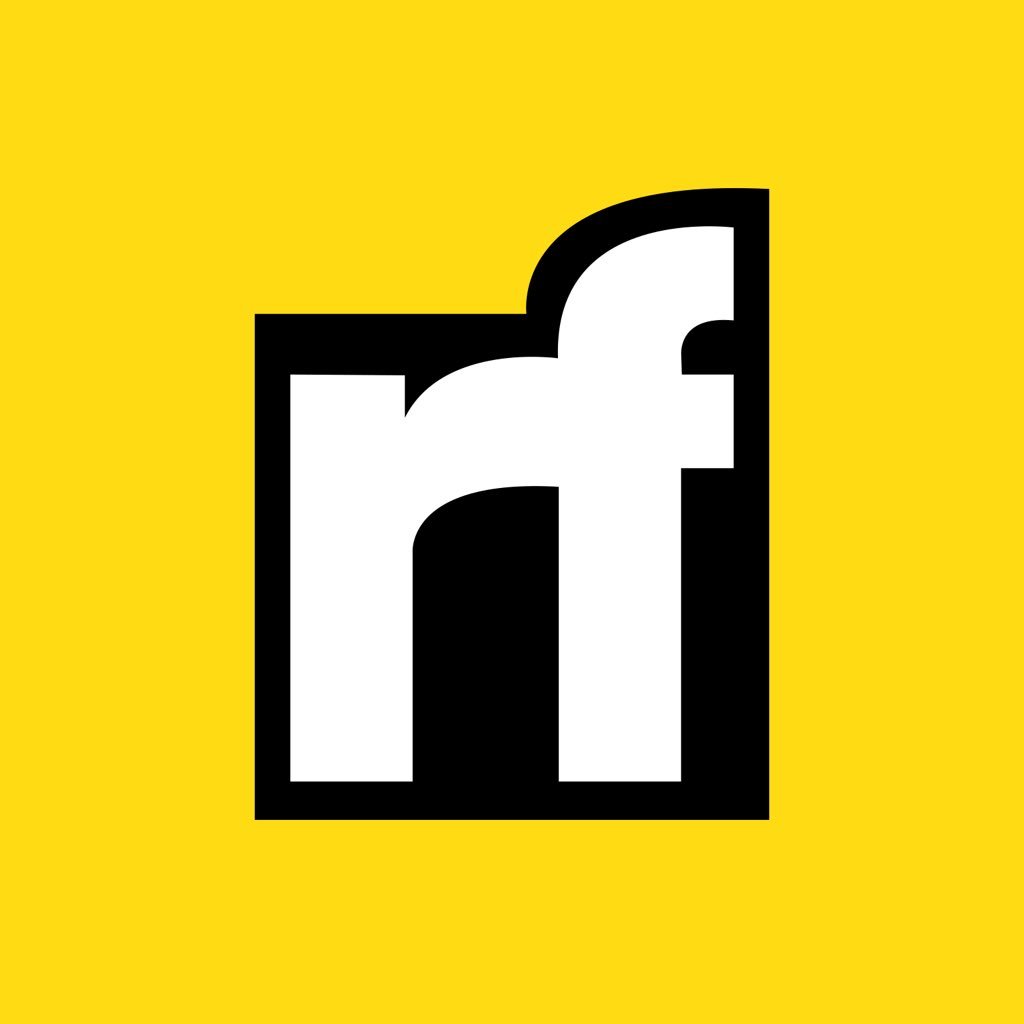
jinesh vora | Sciencx (2024-09-13T11:19:37+00:00) From Data Lakes to Data Mesh: The Emerging Trends of Data Management and Analytics. Retrieved from https://www.scien.cx/2024/09/13/from-data-lakes-to-data-mesh-the-emerging-trends-of-data-management-and-analytics/
Please log in to upload a file.
There are no updates yet.
Click the Upload button above to add an update.