This content originally appeared on HackerNoon and was authored by Kinetograph: The Video Editing Technology Publication
:::info (1) Feng Liang, The University of Texas at Austin and Work partially done during an internship at Meta GenAI (Email: jeffliang@utexas.edu);
(2) Bichen Wu, Meta GenAI and Corresponding author;
(3) Jialiang Wang, Meta GenAI;
(4) Licheng Yu, Meta GenAI;
(5) Kunpeng Li, Meta GenAI;
(6) Yinan Zhao, Meta GenAI;
(7) Ishan Misra, Meta GenAI;
(8) Jia-Bin Huang, Meta GenAI;
(9) Peizhao Zhang, Meta GenAI (Email: stzpz@meta.com);
(10) Peter Vajda, Meta GenAI (Email: vajdap@meta.com);
(11) Diana Marculescu, The University of Texas at Austin (Email: dianam@utexas.edu).
:::
Table of Links
- Abstract and Introduction
- 2. Related Work
- 3. Preliminary
- 4. FlowVid
- 4.1. Inflating image U-Net to accommodate video
- 4.2. Training with joint spatial-temporal conditions
- 4.3. Generation: edit the first frame then propagate
- 5. Experiments
- 5.1. Settings
- 5.2. Qualitative results
- 5.3. Quantitative results
- 5.4. Ablation study and 5.5. Limitations
- Conclusion, Acknowledgments and References
- A. Webpage Demo and B. Quantitative comparisons
5.3. Quantitative results
User study We conducted a human evaluation to compare our method with three notable works: CoDeF [32], Rerender [49], and TokenFlow [13]. The user study involves 25 DAVIS videos and 115 manually designed prompts. Participants are shown four videos and asked to identify which one has the best quality, considering both temporal consistency and text alignment. The results, including the average preference rate and standard deviation from five participants for all methods, are detailed in Table 1. Our method achieved a preference rate of 45.7%, outperforming CoDeF (3.5%), Rerender (10.2%), and TokenFlow (40.4%). During the evaluation, we observed that CoDeF struggles with significant motion in videos. The blurry constructed canonical images would always lead to unsatisfactory results. Rerender occasionally experiences color shifts and bright flickering. TokenFlow sometimes fails to sufficiently alter the video according to the prompt, resulting in an output similar to the original video.
\ Pipeline runtime We also compare runtime efficiency with existing methods in Table 1. Video lengths can vary, resulting in different processing times. Here, we use a video containing 120 frames (4 seconds video with FPS of 30). The resolution is set to 512 × 512. Both our FlowVid model and Rerender [49] use a key frame interval of 4. We generate 31 keyframes by applying autoregressive evaluation twice, followed by RIFE [21] for interpolating the non-key frames.
\
\ The total runtime, including image processing, model operation, and frame interpolation, is approximately 1.5 minutes. This is significantly faster than CoDeF (4.6 minutes), Rerender (10.8 minutes) and TokenFlow (15.8 minutes), being 3.1×, 7.2×, and 10.5 × faster, respectively. CoDeF requires per-video optimization to construct the canonical image. While Rerender adopts a sequential method, generating each frame one after the other, our model utilizes batch processing, allowing for more efficient handling of multiple frames simultaneously. In the case of TokenFlow, it requires a large number of DDIM inversion steps (typically around 500) for all frames to obtain the inverted latent, which is a resource-intensive process. We further report the runtime breakdown (Figure 10) in the Appendix.
\
:::info This paper is available on arxiv under CC 4.0 license.
:::
\
This content originally appeared on HackerNoon and was authored by Kinetograph: The Video Editing Technology Publication
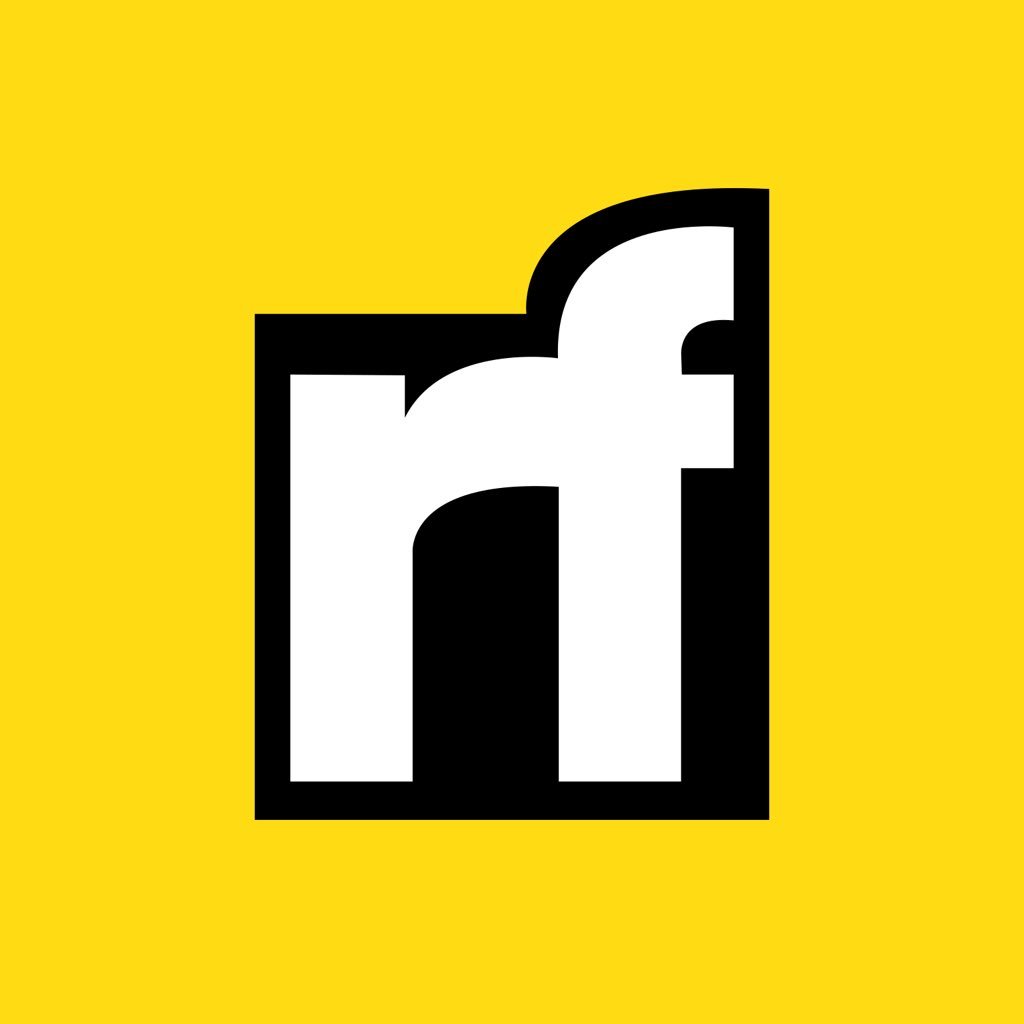
Kinetograph: The Video Editing Technology Publication | Sciencx (2024-10-09T12:45:18+00:00) FlowVid: Taming Imperfect Optical Flows for Consistent Video-to-Video Synthesis: Quantitative result. Retrieved from https://www.scien.cx/2024/10/09/flowvid-taming-imperfect-optical-flows-for-consistent-video-to-video-synthesis-quantitative-result/
Please log in to upload a file.
There are no updates yet.
Click the Upload button above to add an update.