This content originally appeared on DEV Community and was authored by Bekka
Artificial Intelligence has changed the way we consume information, and the way many people research about subjects, projects or even ideas. Since OpenAI introduced its APIs to the public, we've witnessed a profound shift in how people approach learning and productivity, especially in fields like software development. Let's look at how far we've come and possibly what the future holds.
The Past: Foundations of Artificial Intelligence
The roots of AI trace back to Alan Turing's groundbreaking 1950 paper, "Computing Machinery and Intelligence," where he posed the now-famous question, "Can machines think?" This question sparked decades of research and set the stage for developments that would eventually lead to modern AI.
One of the most significant milestones came decades later with Geoffrey Hinton, often dubbed the "Godfather of AI." Hinton’s work in artificial neural networks laid the groundwork for many advancements, establishing neural networks as a key element in modern machine learning.
Breakthroughs: From AlexNet to Generative AI
A pivotal moment in AI's history was the development of AlexNet by Hinton and his team, which significantly advanced image recognition capabilities in 2012. This achievement helped propel the evolution of AI into the era of generative models, enabling machines to not only classify images but also create and simulate realistic content across multiple domains.
For readers interested in understanding more about AlexNet, check out the original research paper here.
Evolution of AI chatbots
As we moved into the 2010s, major tech companies began investing heavily in AI. Google initiated intensive AI research, acquiring Hinton's company, which led to collaborative breakthroughs in machine learning and neural networks. In 2010, DeepMind was founded and later acquired by Google in 2014. OpenAI was established soon after, further pushing the boundaries of what AI could achieve.
These organizations have since led AI innovation, creating popular models like Google’s Gemini and OpenAI’s ChatGPT. These chatbots, driven by advanced neural networks, demonstrate AI’s growing capacity to understand and generate human-like responses, marking a new era in human-computer interaction.
Securing AI: Trusted Execution Environments (TEEs)
As AI models continue to process massive amounts of data, security concerns regarding data privacy have intensified. Trusted Execution Environments (TEEs) offer a solution by providing secure zones within cloud infrastructures. Commonly used in both traditional cloud services and blockchain environments, TEEs protect sensitive information during computation.
A TEE functions as a secure, isolated area within a main processor, ensuring that even privileged users cannot access the data processed within. This hardware-based security layer mitigates the risk of unauthorized access and manipulation, making TEEs a vital component in AI and blockchain security today.
AI x Web3: A New Frontier
With TEEs enabling secure data processing, the integration of AI with Web3 opens up possibilities for creating transparent, autonomous agents.
Combining Web3’s transparency and decentralization with the secure computation capabilities of TEEs, developers can create systems that not only perform tasks autonomously but also uphold user privacy and data integrity. This synergy could redefine trust in AI applications and unlock new possibilities for secure, distributed AI.
Phala Network: The Largest TEE-Powered Blockchain Network
Among TEE solutions, Phala Network stands out as the largest TEE-powered network globally, setting the standard for secure and decentralized data processing. Phala’s blockchain harnesses the unique capabilities of TEE technology to create a secure, private environment for executing AI computations and other complex processes, enabling decentralized applications (dApps) to function securely without sacrificing performance.
Phala Network’s use of TEEs empowers developers to build decentralized applications that prioritize privacy, trust, and security. Unlike traditional cloud-based solutions, Phala’s network ensures that data is handled securely, without reliance on a central authority. This makes it particularly useful for applications that require confidentiality, such as:
Privacy-Preserving AI Agents: Phala supports the development of AI agents that process sensitive data securely and privately, without revealing information to external parties.
Data Marketplaces: Phala enables secure data trading platforms where users can buy and sell data without risking exposure or misuse, enhancing trust in the ecosystem.
Decentralized Finance (DeFi) Privacy: In DeFi, Phala's TEE infrastructure ensures that financial transactions and smart contract data remain confidential, which is crucial for user protection.
Phala Network is pioneering secure AI computation by integrating blockchain's transparency with the robust privacy of TEEs, positioning it as a foundational element for the future of AI and Web3.
The Future: Expanding AI’s Role
Looking ahead, the evolution of AI suggests even greater potential. Future advancements may empower AI to make more sophisticated, context-aware decisions, blending with fields like quantum computing and biotechnology.
Moreover, as regulatory frameworks catch up, we may see policies that enhance transparency and security, encouraging responsible AI development.
This content originally appeared on DEV Community and was authored by Bekka
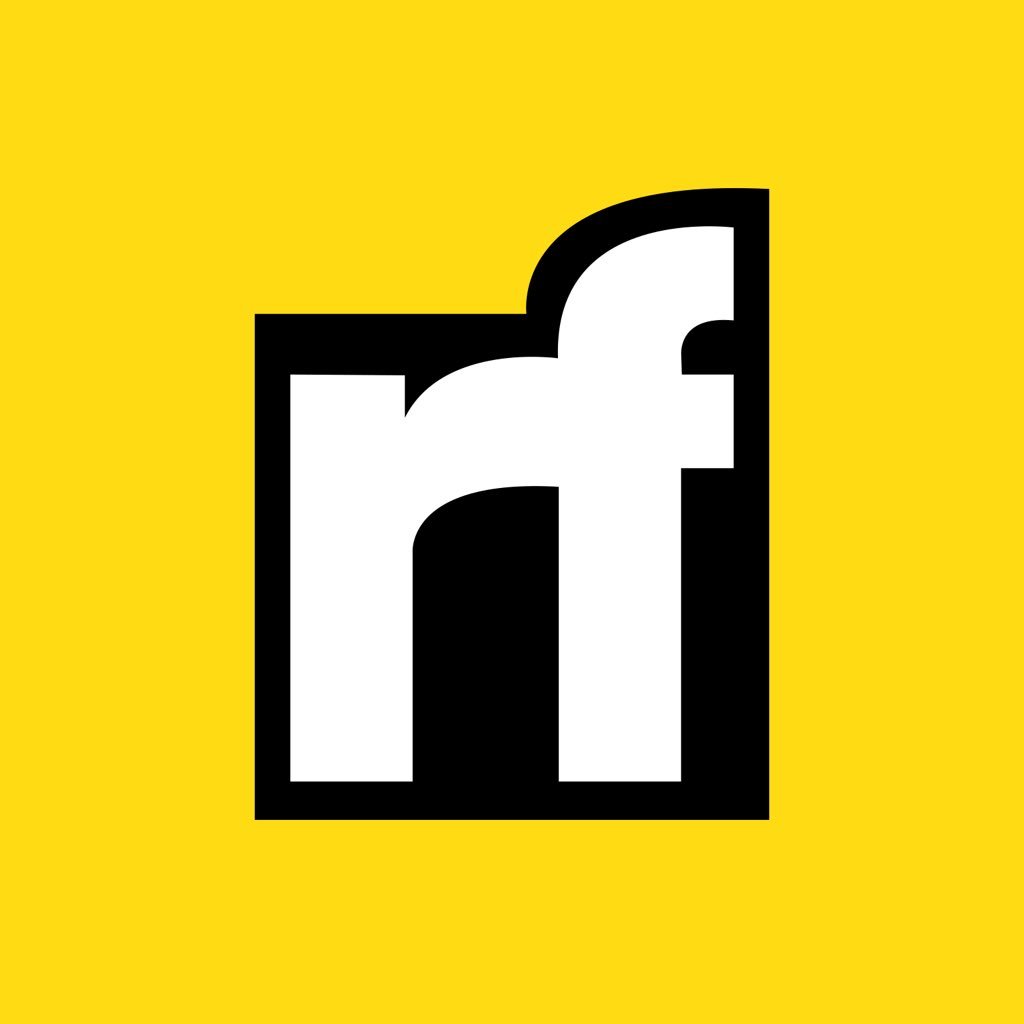
Bekka | Sciencx (2024-10-31T20:49:47+00:00) AI in the 2020s. Retrieved from https://www.scien.cx/2024/10/31/ai-in-the-2020s/
Please log in to upload a file.
There are no updates yet.
Click the Upload button above to add an update.