This content originally appeared on HackerNoon and was authored by Segmentation
:::info Authors:
(1) Zhaoqing Wang, The University of Sydney and AI2Robotics;
(2) Xiaobo Xia, The University of Sydney;
(3) Ziye Chen, The University of Melbourne;
(4) Xiao He, AI2Robotics;
(5) Yandong Guo, AI2Robotics;
(6) Mingming Gong, The University of Melbourne and Mohamed bin Zayed University of Artificial Intelligence;
(7) Tongliang Liu, The University of Sydney.
:::
Table of Links
3. Method and 3.1. Problem definition
3.2. Baseline and 3.3. Uni-OVSeg framework
4. Experiments
6. Broader impacts and References
B. Promptable segmentation.
Evaluation details. We perform a prompt segmentation evaluation on a wide range of datasets in various domains. For the point prompt, we adopt a uniform point grid h×w as input prompts (e.g., 20 × 20). For the box prompt, we use ground-truth bounding boxes as input prompts. 1-pt IoU denotes the oracle performance of one point by evaluating the intersection-overunion (IoU) of the predicted masks that best match ground truth. 1-box IoU denotes is similar to 1-pt IoU. More evaluation results are reported in Fig. 7, Fig. 8 and Fig. 9.
\ Dataset details. A description of each dataset is given in Tab. 5. The iShape dataset has 6 subsets, including antenna, branch, fence, hanger, log and wire.
\
:::info This paper is available on arxiv under CC BY 4.0 DEED license.
:::
\
This content originally appeared on HackerNoon and was authored by Segmentation
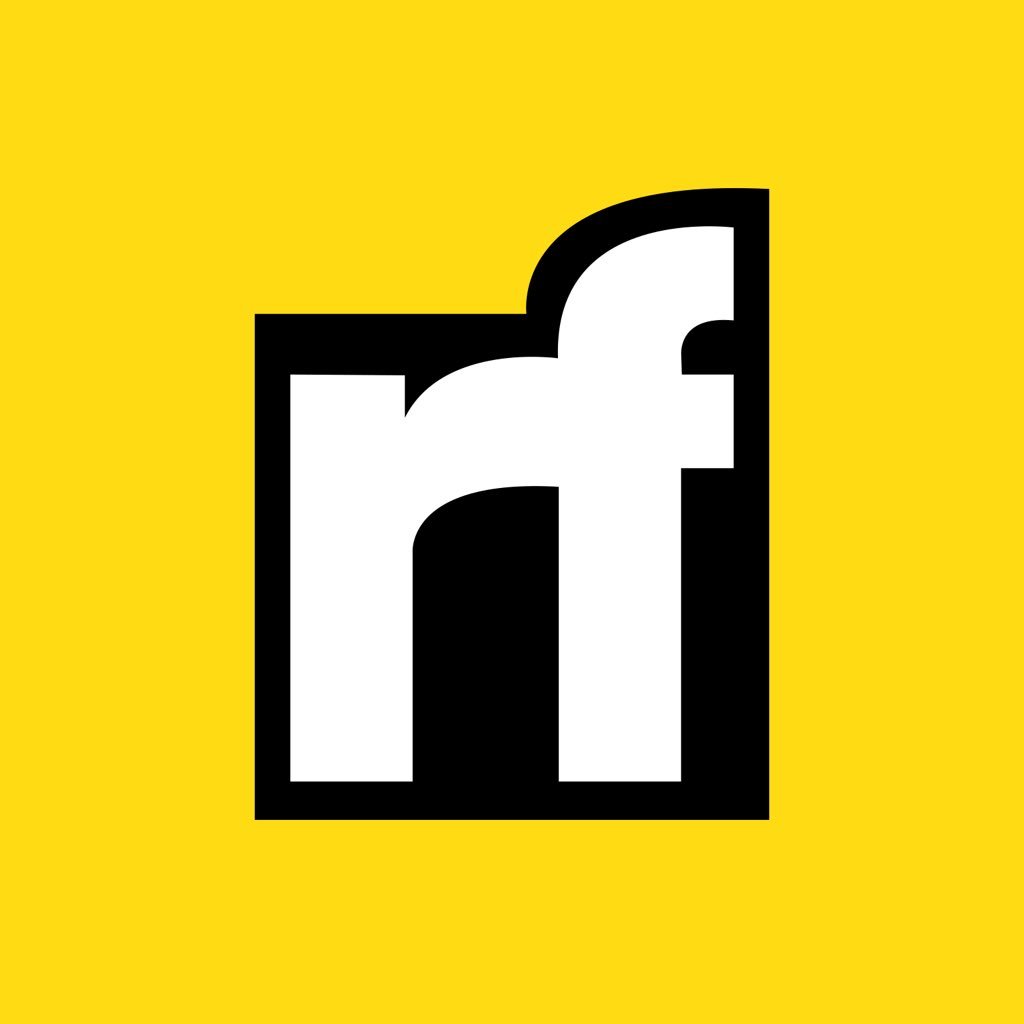
Segmentation | Sciencx (2024-11-13T17:00:27+00:00) Evaluating Promptable Segmentation with Uniform Point Grids and Bounding Boxes on Diverse Datasets. Retrieved from https://www.scien.cx/2024/11/13/evaluating-promptable-segmentation-with-uniform-point-grids-and-bounding-boxes-on-diverse-datasets/
Please log in to upload a file.
There are no updates yet.
Click the Upload button above to add an update.