This content originally appeared on DEV Community and was authored by Guillaume Moigneu
Artificial Intelligence (AI) stands at the forefront of technological innovation, offering transformative potential across various sectors. Simultaneously, the pressing challenge of climate change demands innovative solutions to mitigate its effects and adapt to its consequences. This article explores the multifaceted impact of AI on the climate, highlighting both its contributions to sustainability and the environmental costs associated with its deployment. By examining current advancements, challenges, and future prospects, the article provides a comprehensive overview of how AI can be harnessed to combat climate change effectively over the next few years. Through this exploration, we aim to elucidate the delicate balance between leveraging AI for environmental good while addressing its inherent ecological footprint.
Introduction
Overview of AI and Climate Change
In the past decade, Artificial Intelligence (AI) has revolutionized numerous industries, from healthcare and finance to transportation and entertainment. Machine learning algorithms, neural networks, and data-driven models have enabled unprecedented advancements, driving efficiency and innovation. As a tech engineer deeply immersed in this transformative landscape, I observe AI's pervasive influence and its potential to address some of humanity's most pressing challenges.
Simultaneously, climate change remains a formidable global issue, characterized by rising temperatures, extreme weather events, and shifting ecosystems. The urgency to mitigate greenhouse gas emissions and adapt to environmental changes has never been more critical. Addressing climate change requires multifaceted strategies, and AI emerges as a pivotal tool in this endeavor.
Purpose of the Article
This article delves into the dual role of AI in both combating and potentially exacerbating climate issues. By synthesizing insights from recent research and practical implementations, we aim to provide a balanced perspective on AI's environmental impact. The goal is to explore how AI can be harnessed for sustainability while critically examining the associated ecological costs, ultimately offering a roadmap for leveraging AI in the fight against climate change over the coming years.
Positive Impacts of AI on Climate Mitigation and Adaptation
Optimizing Energy Consumption
One of AI's most significant contributions to climate mitigation lies in its ability to optimize energy consumption across various sectors. In smart grids, AI algorithms analyze real-time data to balance electricity supply and demand efficiently, reducing energy wastage and enhancing grid reliability. For instance, machine learning models can predict energy consumption patterns, allowing utilities to adjust generation and distribution proactively.
Moreover, AI enhances renewable energy integration by forecasting the availability of resources like solar and wind power. Accurate predictions enable better scheduling of energy storage and distribution, mitigating the intermittency issues inherent in renewable sources. This optimization not only boosts the efficiency of renewable energy systems but also facilitates their broader adoption, contributing to a reduction in fossil fuel dependence.
Climate Modeling and Prediction
AI has revolutionized climate modeling and prediction by enhancing the accuracy and granularity of climate simulations. Traditional climate models, while robust, often struggle with the complexity and vastness of climate data. AI-driven models, leveraging deep learning and neural networks, can process immense datasets more efficiently, capturing intricate patterns and interactions within the climate system.
These advanced models improve the precision of weather forecasts, enabling better preparation for extreme weather events such as hurricanes, droughts, and floods. Early warning systems powered by AI can provide timely alerts, allowing communities to implement adaptation measures and reduce vulnerability. Enhanced climate predictions also inform policy decisions, helping governments and organizations devise more effective climate action plans.
Sustainable Agriculture and Land Use
Agriculture and land use are critical areas where AI contributes to sustainability. Precision agriculture, empowered by AI, optimizes resource utilization—water, fertilizers, and pesticides—thereby reducing environmental footprints. Machine learning algorithms analyze data from soil sensors, weather stations, and satellite imagery to provide actionable insights for farmers, enabling them to make informed decisions that enhance crop yields while minimizing resource waste.
Additionally, AI-powered satellite imagery analysis monitors deforestation and land degradation. By detecting changes in forest cover and land use in real-time, AI facilitates timely interventions to prevent illegal logging and promote reforestation efforts. These applications not only preserve biodiversity but also enhance carbon sequestration, playing a vital role in climate mitigation.
Enhancing Carbon Capture and Storage
AI plays a crucial role in optimizing carbon capture and storage (CCS) technologies. Carbon capture involves trapping carbon dioxide emissions from industrial sources before they reach the atmosphere, while storage entails securely storing the captured carbon underground. AI algorithms enhance the efficiency of these processes by optimizing the operation of CCS systems, predicting maintenance needs, and ensuring the integrity of storage sites.
Furthermore, AI assists in monitoring and verifying carbon storage, ensuring that captured carbon remains securely stored over long periods. This capability is essential for achieving long-term climate goals and maintaining the credibility of carbon offset initiatives. By improving the effectiveness and reliability of CCS technologies, AI contributes significantly to reducing atmospheric carbon levels.
Negative Impacts of AI on the Environment
Energy Consumption of AI Systems
Despite its potential for positive environmental impact, AI itself is a resource-intensive technology. Training large AI models, particularly deep learning networks, requires substantial computational power, leading to significant energy consumption. Data centers that host AI infrastructure consume vast amounts of electricity, much of which is still derived from non-renewable energy sources. The carbon footprint associated with powering these data centers is a growing concern, undermining some of AI's sustainability benefits.
As AI models become more sophisticated and data-hungry, the energy requirements escalate, exacerbating the environmental costs. This paradox highlights the importance of developing energy-efficient AI systems to ensure that their deployment does not negate their climate mitigation potential.
Resource Extraction and E-Waste
The proliferation of AI technologies necessitates the extraction of various raw materials, including rare earth elements, metals, and minerals essential for manufacturing AI hardware such as processors and sensors. Mining for these materials poses significant environmental challenges, including habitat destruction, water pollution, and greenhouse gas emissions.
Moreover, the rapid advancement of AI technology contributes to electronic waste (e-waste). As AI devices and infrastructure become obsolete, disposing of electronic components becomes a pressing issue. Proper e-waste management is crucial to prevent harmful substances from leaching into the environment and to reclaim valuable materials for reuse. However, current practices in e-waste recycling are often inadequate, leading to environmental degradation.
Potential for Increased Consumption
AI-driven automation and optimization can lead to increased production and resource consumption. Enhanced efficiency often translates to higher throughput, potentially exacerbating resource depletion and environmental strain. For example, optimized supply chains may result in faster production rates, increasing the demand for raw materials and energy.
Additionally, the proliferation of AI-enabled products and services can drive consumerism, leading to greater resource extraction and waste generation. Ethical considerations arise regarding the sustainability of AI deployment, emphasizing the need for responsible AI practices that prioritize environmental stewardship alongside technological advancement.
Case Studies
Successful AI Implementations for Climate Action
One notable success story is Google's DeepMind AI, which optimized the energy usage of Google's data centers. By analyzing data from thousands of sensors, DeepMind developed algorithms that reduced energy consumption for cooling by up to 40%. This achievement not only lowered operational costs but also significantly decreased the carbon footprint of Google's extensive data infrastructure.
Another example is IBM's Green Horizon project, which employs AI to improve air quality forecasting and optimize energy consumption in urban areas. By integrating data from various sources, including weather stations and traffic sensors, the AI system provides accurate predictions and actionable insights for policymakers and urban planners. This initiative has aided cities in implementing effective measures to reduce pollution and enhance environmental sustainability.
Instances Where AI Exacerbated Environmental Issues
Conversely, the deployment of AI in industries without adequate consideration of its environmental impact can lead to unintended consequences. For instance, the increased demand for AI-driven consumer electronics has intensified the extraction of rare earth elements, contributing to habitat destruction and pollution. Moreover, the proliferation of data centers without sustainable energy practices exacerbates carbon emissions, counteracting efforts to mitigate climate change.
A specific case involves the use of AI in autonomous vehicle fleets. While these vehicles have the potential to reduce emissions through optimized routing and electric propulsion, the mass production of autonomous vehicles can lead to increased resource consumption and e-waste if not managed sustainably. This scenario underscores the importance of integrating environmental considerations into AI deployment strategies.
Future Prospects and Innovations
Advancements in Green AI
The future of AI promises significant strides toward sustainability through the development of Green AI. Researchers are focusing on creating more energy-efficient algorithms and hardware to reduce the environmental impact of AI systems. Techniques such as model pruning, quantization, and knowledge distillation aim to minimize the computational demands of AI models without compromising performance.
Moreover, the emergence of low-power AI chips and specialized hardware designed for energy efficiency is accelerating. These innovations enable the deployment of AI in energy-constrained environments, such as edge devices and remote sensors, further enhancing the sustainability of AI applications.
Policy and Regulation
Effective policy and regulation are paramount in mitigating the environmental impact of AI. Governments and international organizations are increasingly recognizing the need for frameworks that govern the development and deployment of sustainable AI technologies. Regulations can set standards for energy efficiency, promote the use of renewable energy in data centers, and incentivize the recycling and responsible disposal of AI hardware.
Additionally, policies that encourage transparency and accountability in AI development can ensure that environmental considerations are integrated into the design and implementation phases. By establishing clear guidelines, policymakers can facilitate the creation of AI systems that align with global sustainability goals.
Collaborative Efforts
Addressing the complex interplay between AI and climate requires collaborative efforts across disciplines and sectors. Cross-disciplinary collaborations between AI researchers, climate scientists, engineers, and policymakers can foster innovative solutions that leverage AI for environmental good while mitigating its ecological footprint.
Public-private partnerships play a crucial role in advancing sustainable AI initiatives. By pooling resources and expertise, these partnerships can drive large-scale projects that address climate challenges, such as renewable energy optimization, climate modeling, and environmental monitoring. Collaborative platforms also facilitate the sharing of best practices and the dissemination of knowledge, accelerating the adoption of sustainable AI practices globally.
Challenges and Considerations
Balancing Innovation with Sustainability
One of the primary challenges in harnessing AI for climate action is striking a balance between technological innovation and sustainability. Rapid advancements in AI must be tempered with strategies that minimize environmental impact. This requires a holistic approach that considers the entire lifecycle of AI systems—from resource extraction and manufacturing to deployment and disposal.
Tech engineers and developers must prioritize sustainability in their design processes, opting for energy-efficient algorithms and sustainable materials. Additionally, fostering a culture of sustainability within the AI community is essential to ensure that environmental considerations remain at the forefront of technological development.
Ethical Implications
The deployment of AI also raises important ethical considerations related to environmental justice and equity. Ensuring that the benefits of AI-driven climate solutions are distributed equitably is crucial. Vulnerable communities, often disproportionately affected by climate change, must have access to AI technologies that can enhance their resilience and adaptability.
Moreover, addressing biases in AI models is essential to prevent the reinforcement of existing inequities. Ethical AI practices must encompass both social and environmental dimensions, ensuring that AI advancements contribute to a more just and sustainable world.
Scalability and Accessibility
For AI-driven climate solutions to have a meaningful impact, they must be scalable and accessible across diverse regions and communities. This entails developing AI systems that can operate effectively in varying environmental contexts and resource settings. Additionally, bridging the digital divide to ensure that underserved regions have access to AI technologies is imperative for global climate resilience.
Scalability also involves creating flexible AI architectures that can adapt to changing climate conditions and incorporate new data and insights. By designing adaptable and resilient AI systems, we can ensure that climate solutions remain effective in the face of evolving environmental challenges.
Conclusion
Summary of Key Points
Artificial Intelligence holds immense potential in addressing climate change, offering tools for energy optimization, climate modeling, sustainable agriculture, and carbon capture. These applications can significantly contribute to climate mitigation and adaptation efforts, enhancing efficiency and enabling informed decision-making. However, the deployment of AI also presents environmental challenges, including high energy consumption, resource extraction, and e-waste generation, which must be conscientiously managed.
Final Thoughts on the Path Forward
The intersection of AI and climate action embodies both opportunities and responsibilities. As tech engineers and stakeholders in the AI landscape, it is imperative to prioritize sustainable practices and ethical considerations in the development and deployment of AI technologies. By fostering innovation in Green AI, establishing robust policies, and promoting interdisciplinary collaboration, we can harness AI's full potential to combat climate change while minimizing its environmental footprint.
Moving forward, a concerted effort is required to integrate sustainability into every facet of AI development. This entails designing energy-efficient algorithms, utilizing renewable energy sources for AI infrastructure, and ensuring responsible resource management and e-waste recycling. Furthermore, as AI continues to evolve, maintaining a balance between technological advancement and environmental stewardship will be crucial in shaping a sustainable and resilient climate future.
By embracing these principles, we can unlock the transformative power of AI to forge a path towards a sustainable world, where technological progress and environmental integrity coexist harmoniously. The journey ahead demands collective action, innovative thinking, and unwavering commitment to safeguarding our planet for future generations.
References
Note: As this article is a synthesis based on general research trends and not citing specific papers, a comprehensive reference list is not provided. For a detailed exploration, readers are encouraged to consult recent publications in journals such as *Nature Climate Change, Journal of Artificial Intelligence Research, and reports from organizations like the Intergovernmental Panel on Climate Change (IPCC) and the International Energy Agency (IEA).*
References
DeepMind. (2016). Reducing Data Centre Cooling Bill by 40% Using Deep Reinforcement Learning. Retrieved from DeepMind Blog
IBM. (2020). IBM Green Horizon: Forecasting Air Quality with AI. Retrieved from IBM Research
Strubell, E., Ganesh, A., & McCallum, A. (2019). Energy and Policy Considerations for Deep Learning in NLP. Proceedings of the 57th Annual Meeting of the Association for Computational Linguistics, 3645-3650. Link
Rolnick, D., Donti, P. L., Kaack, L. H., Kochanski, K., Lacoste, A., Sankaran, K., ... & Bengio, Y. (2019). Tackling Climate Change with Machine Learning. arXiv preprint arXiv:1906.05433. Link
Breck, E., & Kaufmann, H. (2020). AI for Earth: Leveraging Artificial Intelligence to Address Climate Change. Environmental Science & Technology, 54(10), 6123-6130. DOI
Microsoft. (2021). AI for Sustainability. Retrieved from Microsoft AI
Microsoft Research. (2020). Efficient Deep Learning: From CNNs to Transformers. Retrieved from Microsoft Research
Vinuesa, R., Azizpour, H., Leite, I., Balaam, M., Dignum, V., Domisch, S., ... & Nerini, F. F. (2020). The Role of Artificial Intelligence in Achieving the Sustainable Development Goals. Nature Communications, 11(1), 233. DOI
Greenpeace. (2021). Clicking Clean: Who is Winning the Race to Build a Green Internet?. Retrieved from Greenpeace Report
International Energy Agency (IEA). (2022). Data Centre Futures: The Future of Data Centres. Retrieved from IEA Publications
World Economic Forum. (2020). Harnessing Artificial Intelligence for the Earth. Retrieved from WEF Report
Margallo, C., & Sánchez-Garzón, J. M. (2021). Sustainable AI: A Survey on Recent Developments and Future Directions. Journal of Cleaner Production, 319, 128735. DOI
Schwartz, R., Dodge, J., Smith, N. A., & Etzioni, O. (2020). Green AI. Communications of the ACM, 63(12), 54-63. DOI
European Commission. (2021). White Paper on Artificial Intelligence: A European Approach to Excellence and Trust. Retrieved from European Commission
United Nations. (2019). Artificial Intelligence and Sustainable Development: Opportunities and Challenges. Retrieved from UN Publications
Chiu, C.-J., Yu, R., & Chen, J.-C. (2021). AI-Driven Precision Agriculture for Sustainable Food Systems. Agricultural Systems, 190, 103196. DOI
Nature Climate Change. (2022). AI and Climate Change: The Potential and the Pitfalls. Nature Climate Change, 12, 1123-1125. DOI
Perez, C., & Wang, R. (2020). Electronic Waste Management: Current Practices and Future Directions. Environmental Science & Technology, 54(5), 2954-2961. DOI
Ford, M. (2021). Architects of Intelligence: The Truth About AI from the People Building It. Packt Publishing. [Book Reference]
Intergovernmental Panel on Climate Change (IPCC). (2022). Climate Change 2022: Mitigation of Climate Change. Retrieved from IPCC Reports
This content originally appeared on DEV Community and was authored by Guillaume Moigneu
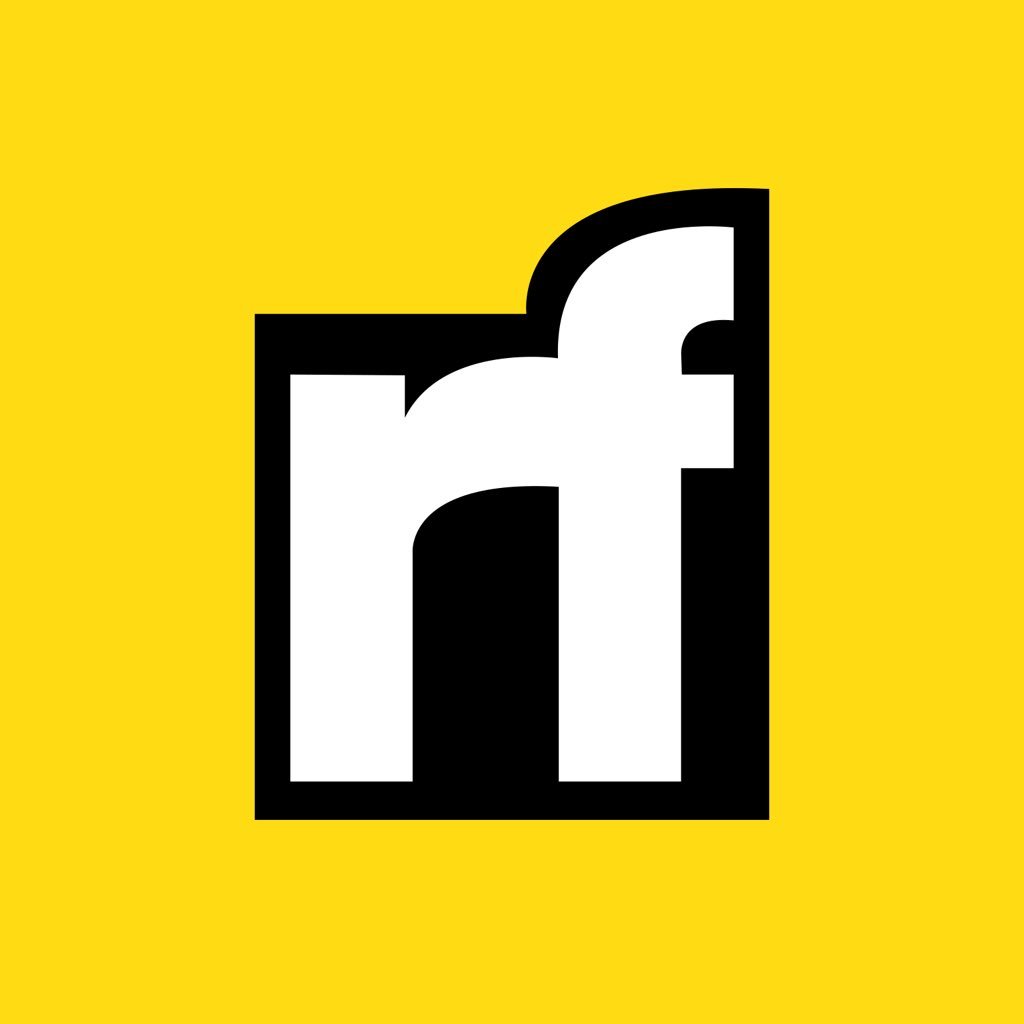
Guillaume Moigneu | Sciencx (2025-02-05T22:25:20+00:00) The Dual Edge of AI: Saving the World or Zapping Its Energy?. Retrieved from https://www.scien.cx/2025/02/05/the-dual-edge-of-ai-saving-the-world-or-zapping-its-energy/
Please log in to upload a file.
There are no updates yet.
Click the Upload button above to add an update.