This content originally appeared on DEV Community and was authored by Mike Young
This is a Plain English Papers summary of a research paper called New AI Training Method Cuts Data Needs in Half While Boosting Performance by 20%. If you like these kinds of analysis, you should join AImodels.fyi or follow us on Twitter.
Overview
- Introduces a new approach for fine-tuning large language models called Selective Self-to-Supervised Fine-Tuning (S2SFT)
- Combines self-supervised and supervised learning to improve model generalization
- Achieves better performance while using less training data
- Reduces catastrophic forgetting during fine-tuning
- Shows significant improvements on multiple benchmark tasks
Plain English Explanation
Selective self-to-supervised fine-tuning works like giving a language model focused practice sessions. Instead of trying to learn everything at once, the model first practices on its ow...
Click here to read the full summary of this paper
This content originally appeared on DEV Community and was authored by Mike Young
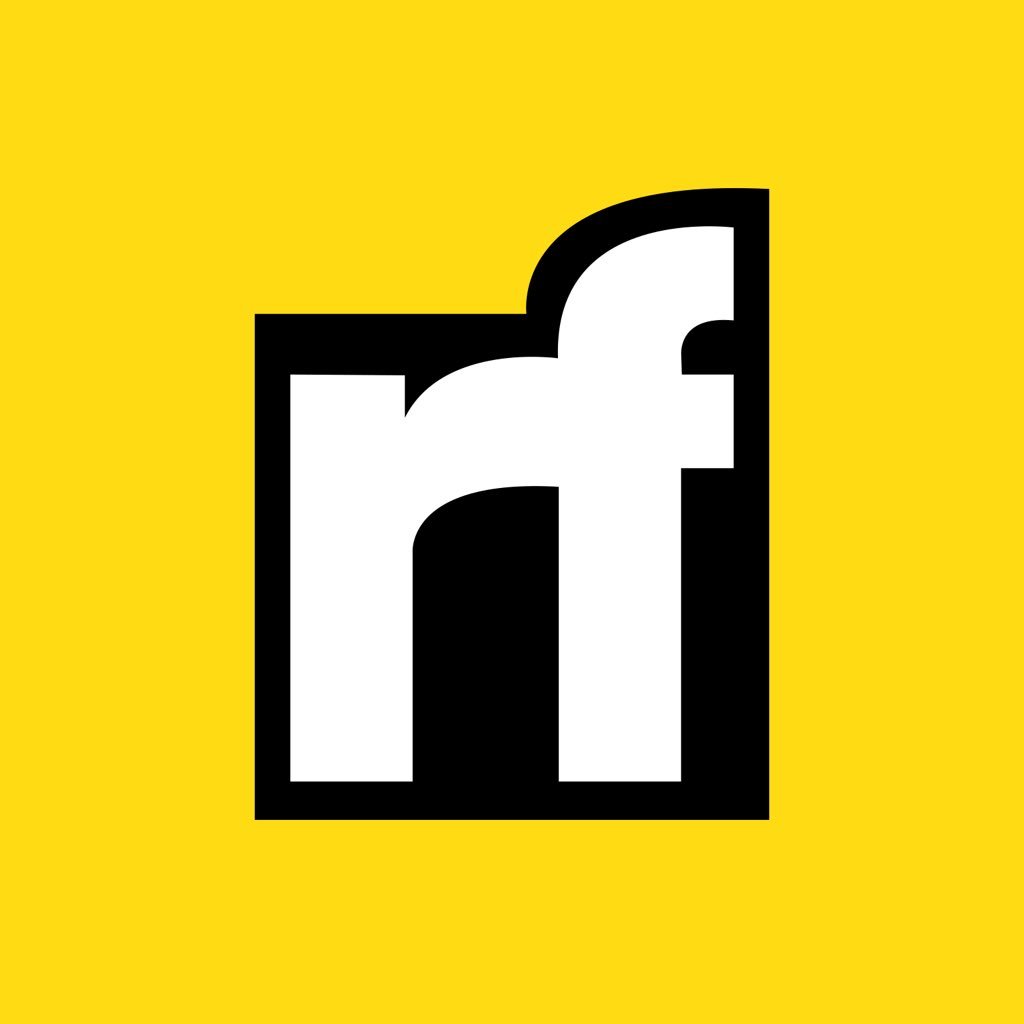
Mike Young | Sciencx (2025-02-18T12:16:33+00:00) New AI Training Method Cuts Data Needs in Half While Boosting Performance by 20%. Retrieved from https://www.scien.cx/2025/02/18/new-ai-training-method-cuts-data-needs-in-half-while-boosting-performance-by-20/
Please log in to upload a file.
There are no updates yet.
Click the Upload button above to add an update.