This content originally appeared on HackerNoon and was authored by Omnidirectional
Table of links
2 BACKGROUND: OMNIDIRECTIONAL 3D OBJECT DETECTION
3.1 Experiment Setup
3.2 Observations
3.3 Summary and Challenges
5 MULTI-BRANCH OMNIDIRECTIONAL 3D OBJECT DETECTION
5.1 Model Design
6.1 Performance Prediction
5.2 Model Adaptation
6.2 Execution Scheduling
8.1 Testbed and Dataset
8.2 Experiment Setup
8.3 Performance
8.4 Robustness
8.5 Component Analysis
8.6 Overhead
7 IMPLEMENTATION
We implemented Panopticus using Python and CUDA for GPU-based acceleration. All neural networks were developed using PyTorch [41] and trained on the training set in the nuScenes dataset [2]. Note that Panopticus is compatible with other 3D perception datasets such as Waymo [46], having sensor configurations similar to nuScenes. The neural networks for 3D object detection are developed based on
\
Figure 9: Setup for mobile testbed and dataset. MMDetection3D [35]. For the camera motion network, we customized and trained [53] to produce consistent relative poses between two consecutive frames for any given camera view. For the object tracker, we modified SimpleTrack [36] to use velocity for 3D Kalman’s state transition model. We used the GPU-accelerated XGBoost library [12] and linear model in scikit-learn [43] for performance predictors, and PuLP [17] for the ILP solver. In the model adaptation stage, memory usage is profiled via tegrastats
\ We optimized the performance of our multi-branch model in various ways. We used TensorRT [11] to modularize the neural networks and to accelerate the inference. Networks converted to TensorRT are optimized using floating-point 16 (FP16) quantization and layer fusion, etc. Camera view images assigned to the same networks, such as backbone network or DepthNet, are batch-processed together. Additionally, we used CUDA Multi-Stream [5] to process these multiple networks in parallel on an edge GPU. Meanwhile, we noticed an accuracy loss due to the simplistic modularization of our multi-branch model. Specifically, DepthNets and BEV head trained with a specific backbone network are incompatible with others. One-size-fits-all DepthNet is not practical since each backbone generates a 2D feature map of different sizes. Thus, our model includes DepthNet variants tailored to each backbone, ensuring accurate depth estimation. In contrast, the BEV head takes inputs of a consistent shape, regardless of the preceding networks—backbones and DepthNets. This allows us to train a universal BEV head compatible with any combination of preceding networks. We trained the BEV head from scratch, while the pre-trained backbones and DepthNets were fine-tuned.
:::info Authors:
:::
:::info This paper is available on arxiv under CC by 4.0 Deed (Attribution 4.0 International) license.
:::
\
This content originally appeared on HackerNoon and was authored by Omnidirectional
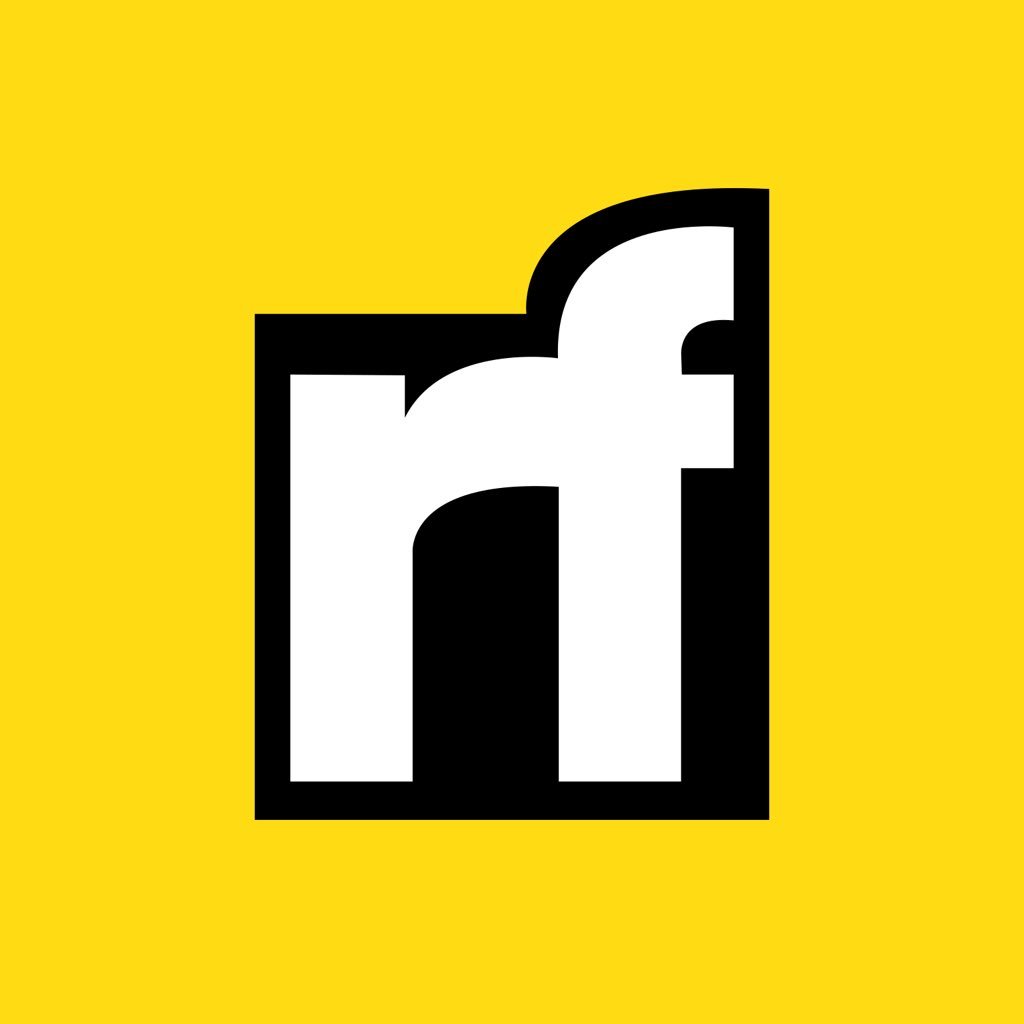
Omnidirectional | Sciencx (2025-03-02T21:48:15+00:00) How Panopticus Uses AI to Detect Objects in 3D. Retrieved from https://www.scien.cx/2025/03/02/how-panopticus-uses-ai-to-detect-objects-in-3d/
Please log in to upload a file.
There are no updates yet.
Click the Upload button above to add an update.