This content originally appeared on DEV Community and was authored by Midas/XIV
- Flower is the framework to do distributed machine learning training jobs in a privacy-enhancing way.
- For some reason if all data cannot be shared, you would have to train a model in a distributed way without having to collect all the data centrally. Instead of moving data to the training process, you can move the training to the data in different centers, then you can collect all the model parameters and centralize it, not the raw data itself. this way you can benefit from all the data across all hospitals without ever needing to raw data to leave any place.
Why FL
data is naturally distributed, for example in governments data is distributed across many governments. Data might be sensitive, volume of data might be too high, regulations on data, data practicality.
When a model is trained without certain datatypes, it not only fails to predict the scenario correctly, but predicts it incorrectly which may be harmful.
it is impossible to centralize data, a large amount of data is unused as it is sensitive.
How does a federated tuning system works.
architecture; global model => server => clients.
server there's is usually no data, but there can be test data to check global models
global model sends initial parameter to server which passes it to all the clients.
role of server is to coordinate the training on the clients,
FL Algorithm
initialization:
server initializes the global model.Communication round:
for each communication roundServer sends the global model to participating clients
Each client receives the global model.
Client training and model update:
for each participating , client trains on local data, client sends it's locally updated model to the server.Model Aggregation
Server aggregates the models received from all clients using aggregation algorithm ( for fedAvg [strategy])Convergence Check
if convergence criteria are met, then we end this process else we go again.
federated learning is an iterative process, clients train the model and the server aggregates model updates.
How to tune a federated system.
[entire chapter was for configuring the server and client, the hyper parameters you can pass to the flower framework.]
A common way to aggregate the model, is to average the weights.
what's data privacy in federated learning.
differential privacy ?
Adding minor noise to a sensitive dataset which could potentially be in the dataset.
This content originally appeared on DEV Community and was authored by Midas/XIV
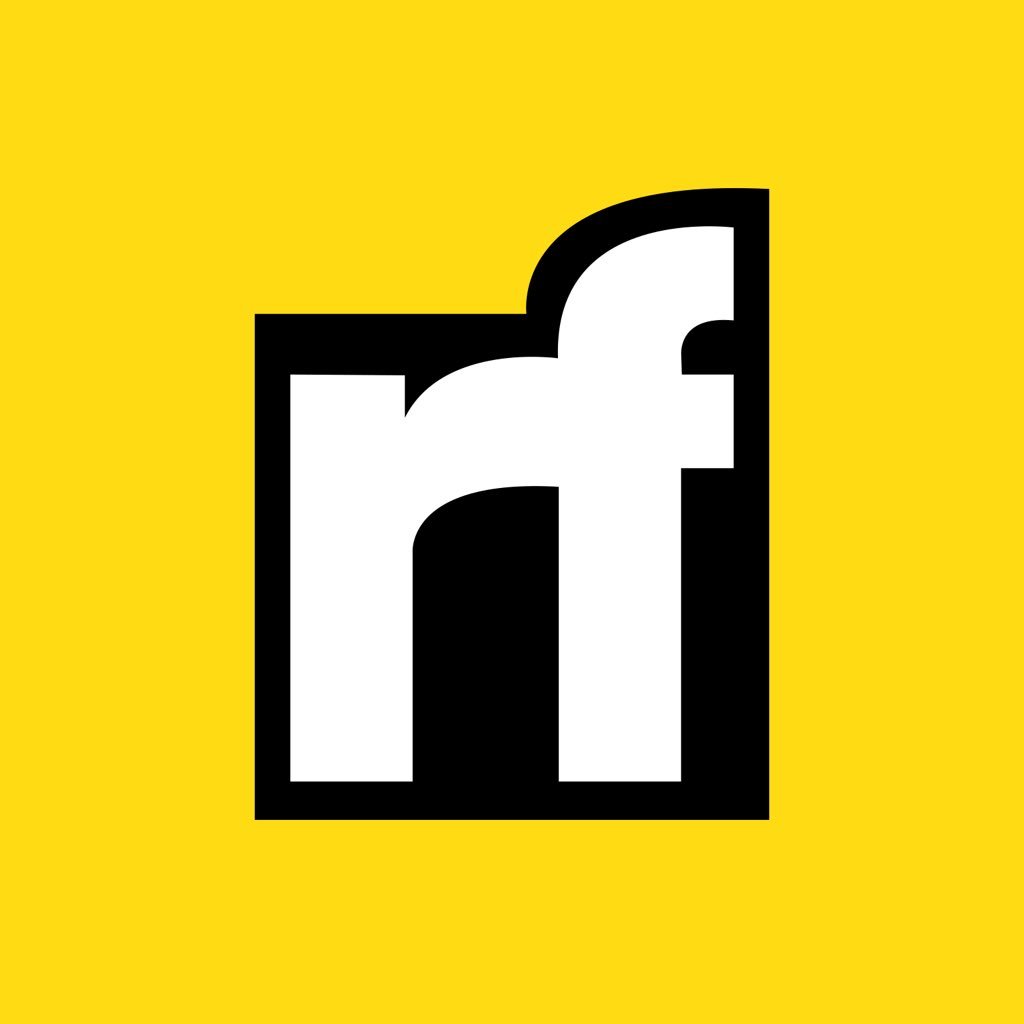
Midas/XIV | Sciencx (2024-07-30T19:45:08+00:00) Federated Learning. Retrieved from https://www.scien.cx/2024/07/30/federated-learning/
Please log in to upload a file.
There are no updates yet.
Click the Upload button above to add an update.