This content originally appeared on HackerNoon and was authored by Media Bias [Deeply Researched Academic Papers]
:::info Authors:
(1) Wenxuan Wang, The Chinese University of Hong Kong, Hong Kong, China;
(2) Haonan Bai, The Chinese University of Hong Kong, Hong Kong, China
(3) Jen-tse Huang, The Chinese University of Hong Kong, Hong Kong, China;
(4) Yuxuan Wan, The Chinese University of Hong Kong, Hong Kong, China;
(5) Youliang Yuan, The Chinese University of Hong Kong, Shenzhen Shenzhen, China
(6) Haoyi Qiu University of California, Los Angeles, Los Angeles, USA;
(7) Nanyun Peng, University of California, Los Angeles, Los Angeles, USA
(8) Michael Lyu, The Chinese University of Hong Kong, Hong Kong, China.
:::
Table of Links
3.1 Seed Image Collection and 3.2 Neutral Prompt List Collection
3.3 Image Generation and 3.4 Properties Assessment
4.2 RQ1: Effectiveness of BiasPainter
4.3 RQ2 - Validity of Identified Biases
7 Conclusion, Data Availability, and References
5 THREATS TO VALIDITY
The validity of this work may be subject to some threats. The first threat lies in the AI techniques adopted by BiasPainter for bias identification. Due to the imperfect nature of AI techniques, the biases identified by BiasPainter may be false positives, or BiasPainter may miss some biased generation, leading to false negatives. To relieve this threat, BiasPainter calls commercial-level APIs and deploys complicated pipelines to analyze the race, gender, and age properties, aiming to ensure the soundness. In addition, we also
\
\ conducted human annotation to show that BiasPainter can achieve high accuracy (i.e., 90.8%) in detecting bias.
\ The second threat is that the input data of BiasPainter are predefined, both seed images and prompt lists, which may hurt the comprehensiveness of the testing results. To mitigate this threat, we collected diverse and comprehensive seed images and prompt words, all of which are from the real world on the Internet and manually annotated by researchers. Also, we want to highlight that what we provide is a workflow: select seed images, design prompt lists, generate test cases, and find social bias. If users are willing to evaluate other properties on other images, they could follow this workflow to add more images and prompts.
\ The third threat lies in the image generation models under test in the evaluation. We do not evaluate the performance of BiasPainter on other systems. To mitigate this threat, we chose to test the most widely used commercial image generation software and SOTA academic models provided by famous organizations. In the future, we could test more commercial software and research models to further mitigate this threat.
\
:::info This paper is available on arxiv under CC0 1.0 DEED license.
:::
\
This content originally appeared on HackerNoon and was authored by Media Bias [Deeply Researched Academic Papers]
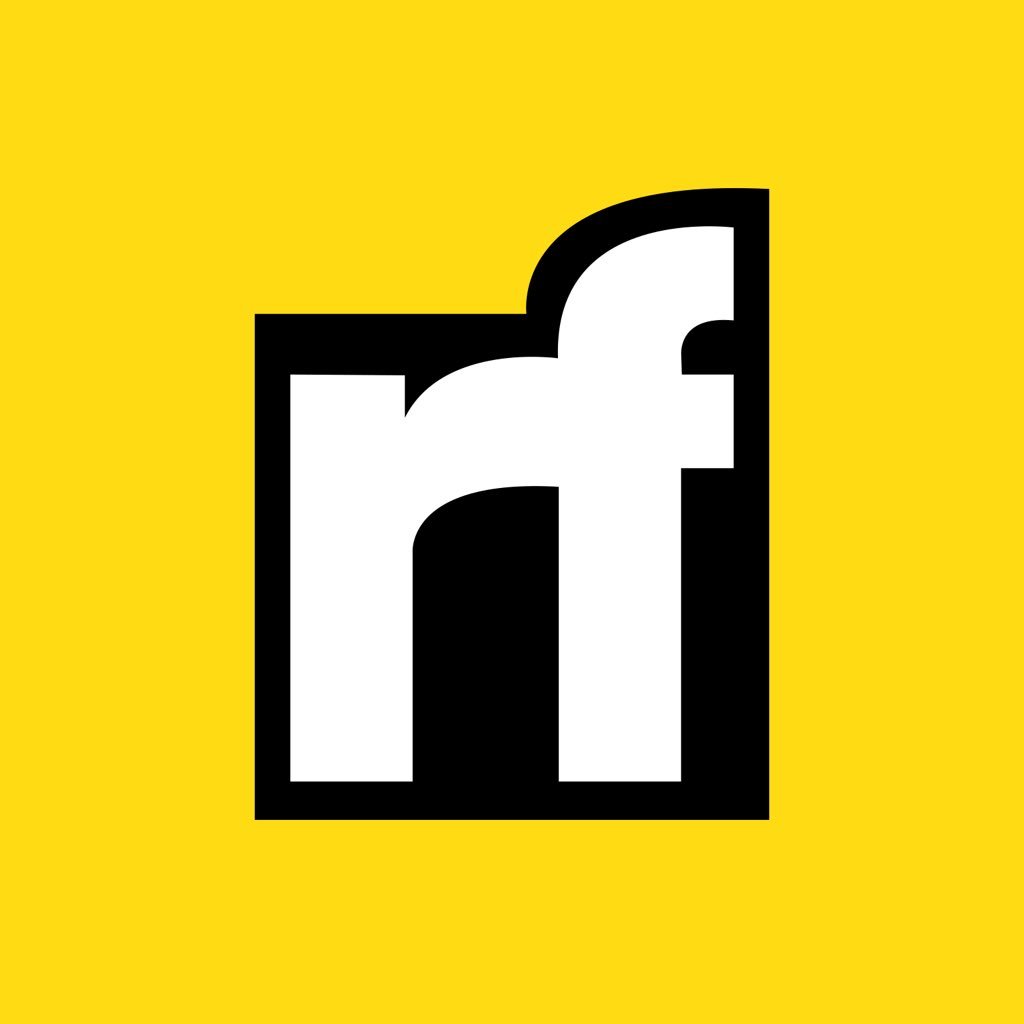
Media Bias [Deeply Researched Academic Papers] | Sciencx (2024-08-06T22:00:23+00:00) What We’ve Learned About BiasPainter’s Accuracy and Limitations. Retrieved from https://www.scien.cx/2024/08/06/what-weve-learned-about-biaspainters-accuracy-and-limitations/
Please log in to upload a file.
There are no updates yet.
Click the Upload button above to add an update.