This content originally appeared on HackerNoon and was authored by Tech Media Bias [Research Publication]
Table of Links
3 End-to-End Adaptive Local Learning
3.1 Loss-Driven Mixture-of-Experts
3.2 Synchronized Learning via Adaptive Weight
4 Debiasing Experiments and 4.1 Experimental Setup
4.3 Ablation Study
4.4 Effect of the Adaptive Weight Module and 4.5 Hyper-parameter Study
6 Conclusion, Acknowledgements, and References
3 End-to-End Adaptive Local Learning
To debias, we propose the end-To-end Adaptive Local Learning (TALL) framework, shown in Figure 2. To address the discrepancy modeling problem, this framework integrates a loss-driven Mixture-of-Experts module to adaptively provide customized models for different users by an end-to-end learning procedure. To address the unsynchronized learning problem, the framework involves an adaptive weight module to synchronize the learning paces of different users by adaptively adjusting weights in the objective function.
\
\
:::info Authors:
(1) Jinhao Pan [0009 −0006 −1574 −6376], Texas A&M University, College Station, TX, USA;
(2) Ziwei Zhu [0000 −0002 −3990 −4774], George Mason University, Fairfax, VA, USA;
(3) Jianling Wang [0000 −0001 −9916 −0976], Texas A&M University, College Station, TX, USA;
(4) Allen Lin [0000 −0003 −0980 −4323], Texas A&M University, College Station, TX, USA;
(5) James Caverlee [0000 −0001 −8350 −8528]. Texas A&M University, College Station, TX, USA.
:::
:::info This paper is available on arxiv under CC BY 4.0 DEED license.
:::
\
This content originally appeared on HackerNoon and was authored by Tech Media Bias [Research Publication]
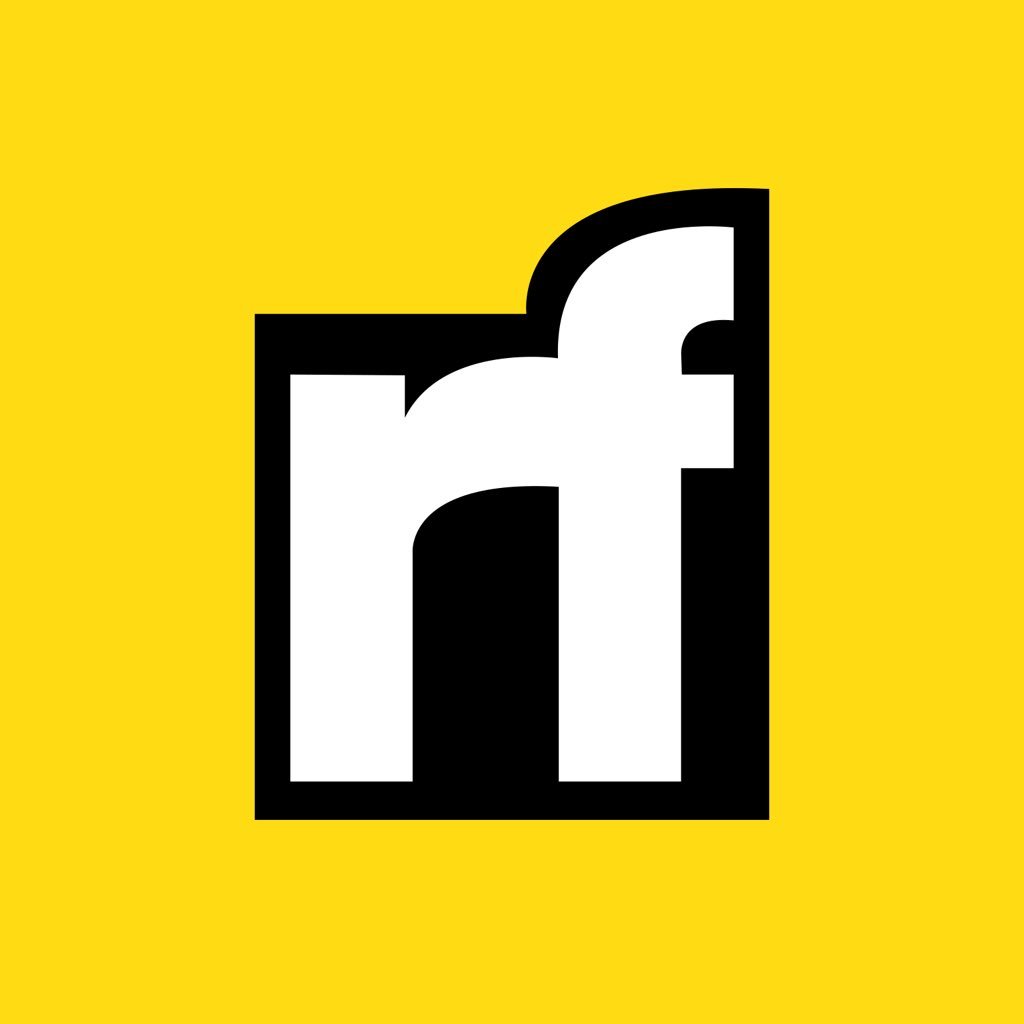
Tech Media Bias [Research Publication] | Sciencx (2024-08-21T13:00:15+00:00) Countering Mainstream Bias via End-to-End Adaptive Local Learning: Adaptive Local Learning. Retrieved from https://www.scien.cx/2024/08/21/countering-mainstream-bias-via-end-to-end-adaptive-local-learning-adaptive-local-learning/
Please log in to upload a file.
There are no updates yet.
Click the Upload button above to add an update.