This content originally appeared on HackerNoon and was authored by The FewShot Prompting Publication
:::info Authors:
(1) Sebastian Dziadzio, University of Tübingen (sebastian.dziadzio@uni-tuebingen.de);
(2) Çagatay Yıldız, University of Tübingen;
(3) Gido M. van de Ven, KU Leuven;
(4) Tomasz Trzcinski, IDEAS NCBR, Warsaw University of Technology, Tooploox;
(5) Tinne Tuytelaars, KU Leuven;
(6) Matthias Bethge, University of Tübingen.
:::
Table of Links
2. Two problems with the current approach to class-incremental continual learning
3. Methods and 3.1. Infinite dSprites
4. Related work
4.1. Continual learning and 4.2. Benchmarking continual learning
5.1. Regularization methods and 5.2. Replay-based methods
5.4. One-shot generalization and 5.5. Open-set classification
Conclusion, Acknowledgments and References
5.6. Online vs. offline
In all previous experiments, we applied our method in batch mode: we performed multiple training passes over the data for each task. However, efficiently learning from streaming data might require observing each training sample only once to make sure computation is not becoming a bottleneck. This is why we test our method in the online learning regime and compare it to two batch learning scenarios. The results are shown in Fig. 9. Unsurprisingly, training for multiple epochs results in better and more robust accuracy on past tasks; it is however worth noting that our method still improves over time in the online learning scenario. It is possible that, given enough tasks, all three curves would converge.
\
\
\
:::info This paper is available on arxiv under CC 4.0 license.
:::
\
This content originally appeared on HackerNoon and was authored by The FewShot Prompting Publication
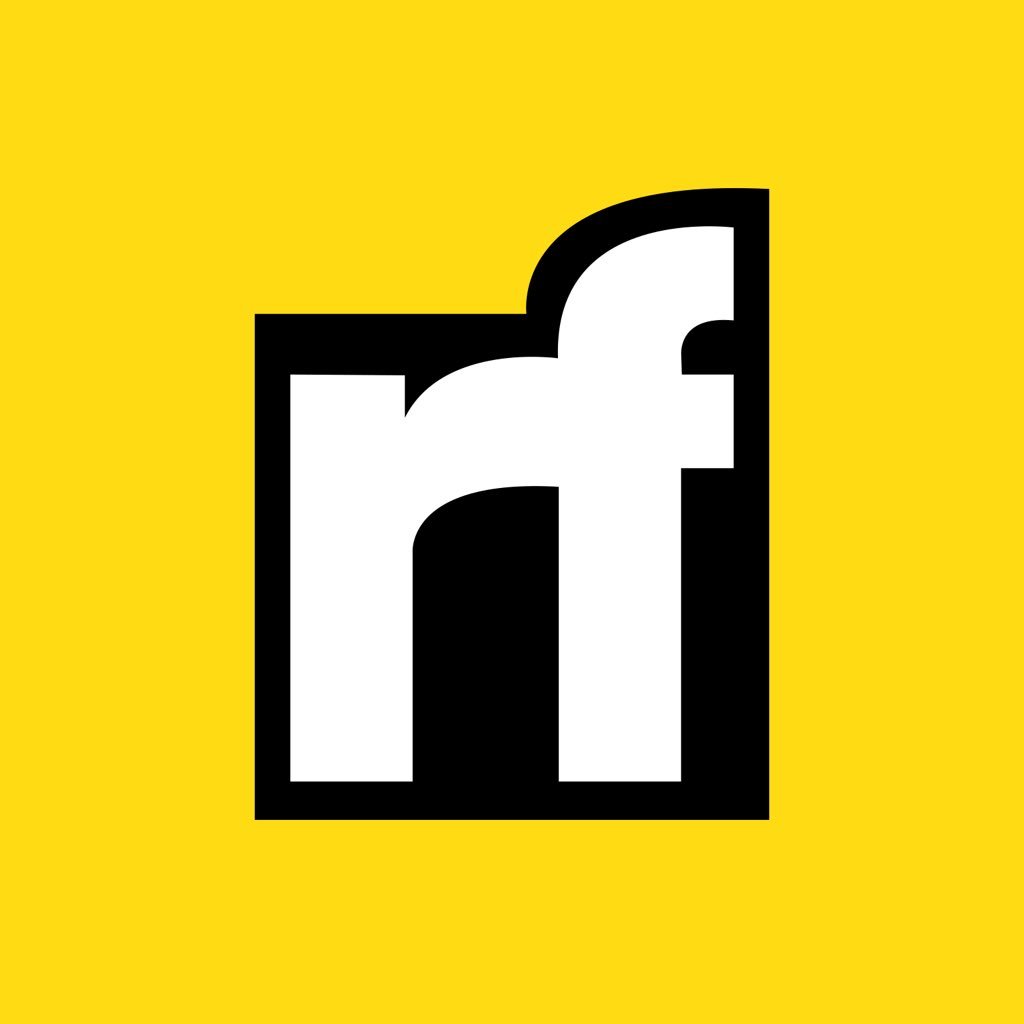
The FewShot Prompting Publication | Sciencx (2024-08-27T23:00:27+00:00) Batch Training vs. Online Learning. Retrieved from https://www.scien.cx/2024/08/27/batch-training-vs-online-learning/
Please log in to upload a file.
There are no updates yet.
Click the Upload button above to add an update.