This content originally appeared on DEV Community and was authored by Furkan Gözükara
I have done an extensive multi-GPU FLUX Full Fine Tuning / DreamBooth training experimentation on RunPod by using 2x A100–80 GB GPUs (PCIe) since this was commonly asked of me.
Image 1
-
Image 1 shows that only first part of installation of Kohya GUI took 30 minutes on a such powerful machine on a very expensive Secure Cloud pod — 3.28 USD per hour
-
There was also part 2, so just installation took super time
-
On Massed Compute, it would take like 2–3 minutes
-
This is why I suggest you to use Massed Compute over RunPod, RunPod machines have terrible hard disk speeds and they are like lottery to get good ones
Image 2, 3 and 4
-
Image 2 shows speed of our very best config FLUX Fine Tuning training shared below when doing 2x Multi GPU training
-
Used config name is : Quality_1_27500MB_6_26_Second_IT.json
-
Image 3 shows VRAM usage of this config when doing 2x Multi GPU training
-
Image 4 shows the GPUs of the Pod
Image 5 and 6
-
Image 5 shows speed of our very best config FLUX Fine Tuning training shared below when doing a single GPU training
-
Used config name is : Quality_1_27500MB_6_26_Second_IT.json
-
Image 6 shows this setup used VRAM amount
Image 7 and 8
-
Image 7 shows speed of our very best config FLUX Fine Tuning training shared below when doing a single GPU training and Gradient Checkpointing is disabled
-
Used config name is : Quality_1_27500MB_6_26_Second_IT.json
-
Image 8 shows this setup used VRAM amount
Image 9 and 10
-
Image 9 shows speed of our very best config FLUX Fine Tuning training shared below when doing 2x Multi GPU training — this time Fused Backward Pass is disabled
-
Used config name is : Quality_1_27500MB_6_26_Second_IT.json
-
Image 10 shows this setup used VRAM amount
Image 11
-
Image 2 shows speed of our very best config FLUX Fine Tuning training shared below when doing 2x Multi GPU training on a different Pod
-
Used config name is : Quality_1_27500MB_6_26_Second_IT.json
Conclusions
-
For multi-GPU FLUX Fine Tuning, you have to use at least 80 GB GPUs
-
When doing multi-GPU FLUX Fine Tuning, Fused Backward Pass brings 0 VRAM usage improvements but slows down the training — I am going to report this
-
With A100 GPU, you are able to reach 2.89 second / it — probably it will get better as you do more steps
-
With 2x A100 GPU, you are able to reach 4.1 second / it — effective speed 2.05 second / it
-
The speed gain is 0.75 / 2.9 = 26% — so 2x GPU training totally doesn’t worth it at the moment
-
If speed drop stays same due to multi-GPU overhead, 8x A100 may be beneficial but you have to experiment it properly and calculate speed gain
-
Currently single L40S would be way cheaper and faster
-
When doing multi-GPU FLUX LoRA training, we almost gain linear speed increase — I have tested with 8x RTX A6000 : https://www.patreon.com/posts/110879657
-
As shown in Image 11, there is also a chance that you will get a way worse performing pod
-
With same 2x A100 GPU and no visible difference, that random pod performed 1/4 speed of another same config pod — terrible terrible speed
This content originally appeared on DEV Community and was authored by Furkan Gözükara
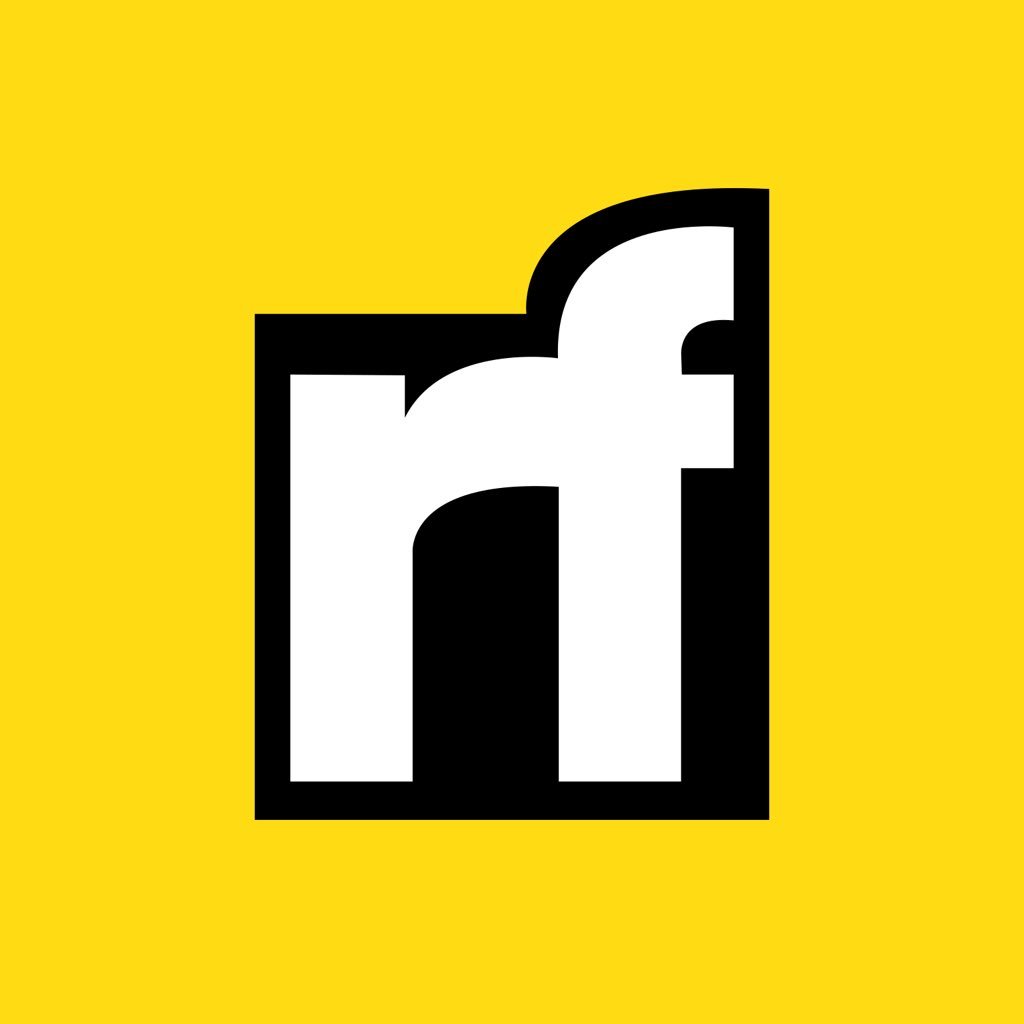
Furkan Gözükara | Sciencx (2024-09-21T16:42:16+00:00) Multi-GPU FLUX Full Fine Tuning Experiments and Requirements on RunPod and Conclusions. Retrieved from https://www.scien.cx/2024/09/21/multi-gpu-flux-full-fine-tuning-experiments-and-requirements-on-runpod-and-conclusions/
Please log in to upload a file.
There are no updates yet.
Click the Upload button above to add an update.