This content originally appeared on HackerNoon and was authored by Auto Encoder: How to Ignore the Signal Noise
:::info Authors:
(1) Tony Lee, Stanford with Equal contribution;
(2) Michihiro Yasunaga, Stanford with Equal contribution;
(3) Chenlin Meng, Stanford with Equal contribution;
(4) Yifan Mai, Stanford;
(5) Joon Sung Park, Stanford;
(6) Agrim Gupta, Stanford;
(7) Yunzhi Zhang, Stanford;
(8) Deepak Narayanan, Microsoft;
(9) Hannah Benita Teufel, Aleph Alpha;
(10) Marco Bellagente, Aleph Alpha;
(11) Minguk Kang, POSTECH;
(12) Taesung Park, Adobe;
(13) Jure Leskovec, Stanford;
(14) Jun-Yan Zhu, CMU;
(15) Li Fei-Fei, Stanford;
(16) Jiajun Wu, Stanford;
(17) Stefano Ermon, Stanford;
(18) Percy Liang, Stanford.
:::
Table of Links
Author contributions, Acknowledgments and References
3 Aspects
We evaluate 12 diverse aspects crucial for deploying text-to-image models, as detailed in Table 1.
\
\
\ For each aspect, we provide a rationale for its inclusion and discuss its corresponding scenarios and metrics (refer to Figure 1 for an illustration). Further details regarding all scenarios and metrics will be presented in §4 and §5.
\ Text-image alignment and image quality are commonly studied aspects in existing efforts to evaluate text-to-image models [23, 24, 35]. Since these are general aspects, we can assess these aspects for any scenario. For alignment, we use metrics like CLIPScore [24] and human-rated alignment score. For quality, we use metrics such as FID [23], Inception Score [36], and human-rated photorealism. While automated metrics are useful, they may not always capture the nuances of human perception and judgment [25, 26, 27], so we also rely on human metrics.
\ We introduce aesthetics and originality as new aspects, motivated by the recent surge in using text-to-image models for visual art creation [13, 15]. In particular, originality is crucial for addressing
\
\ copyright infringement concerns in generative AI [37, 38, 39]. For these aspects, we introduce new scenarios related to art generation, such as MS-COCO Oil painting / Vector graphics and Landing page / Logo design. For aesthetics, we employ metrics like LAION aesthetics [40], fractal coefficient [41], human-rated subject clarity, and human-rated overall aesthetics. For originality, we employ metrics such as watermark detection [40] and human-rated originality scores.
\ Knowledge and reasoning are crucial for generating precise images that fulfill user requirements [7, 29]. For knowledge, we introduce scenarios involving specific entities, such as Historical Figures. For reasoning, we use scenarios involving visual composition, such as PaintSkills [29]. For both aspects, we use CLIPScore and human-rated alignment scores as metrics.
\ Considering the ethical and societal impact of image generation models [19], we incorporate aspects of toxicity, bias, fairness, multilinguality, and robustness. Our definitions, outlined in Table 1, align with [1]. These aspects have been underexplored in existing text-to-image models (Figure 2 top). However, these aspects are crucial for real-world model deployment. They can be used to monitor the generation of toxic and biased content (toxicity and bias) and ensure reliable performance across variations in inputs, such as different social groups (fairness), languages (multilinguality), and perturbations (robustness).
\ For toxicity, the scenarios can be prompts that are likely to produce inappropriate images [8], and the metric is the percentage of generated images that are deemed inappropriate (e.g., NSFW, nude, or blacked out). For bias, the scenarios can be prompts that may trigger stereotypical associations [33], and the metrics are the demographic biases in generated images, such as gender bias and skin tone bias. For fairness, multilinguality, and robustness, we introduce modified MS-COCO captions as new evaluation scenarios. Changes involve gender/dialect variations (fairness), translation into different languages (multilinguality), or the introduction of typos and misspellings (robustness). We then measure the performance change (e.g., CLIPScore) compared to the unmodified MS-COCO scenario.
\ Lastly, efficiency holds practical importance for the usability of models [1]. Inference time serves as the metric, and any scenarios can be employed, as efficiency is a general aspect.
:::info This paper is available on arxiv under CC BY 4.0 DEED license.
:::
\
This content originally appeared on HackerNoon and was authored by Auto Encoder: How to Ignore the Signal Noise
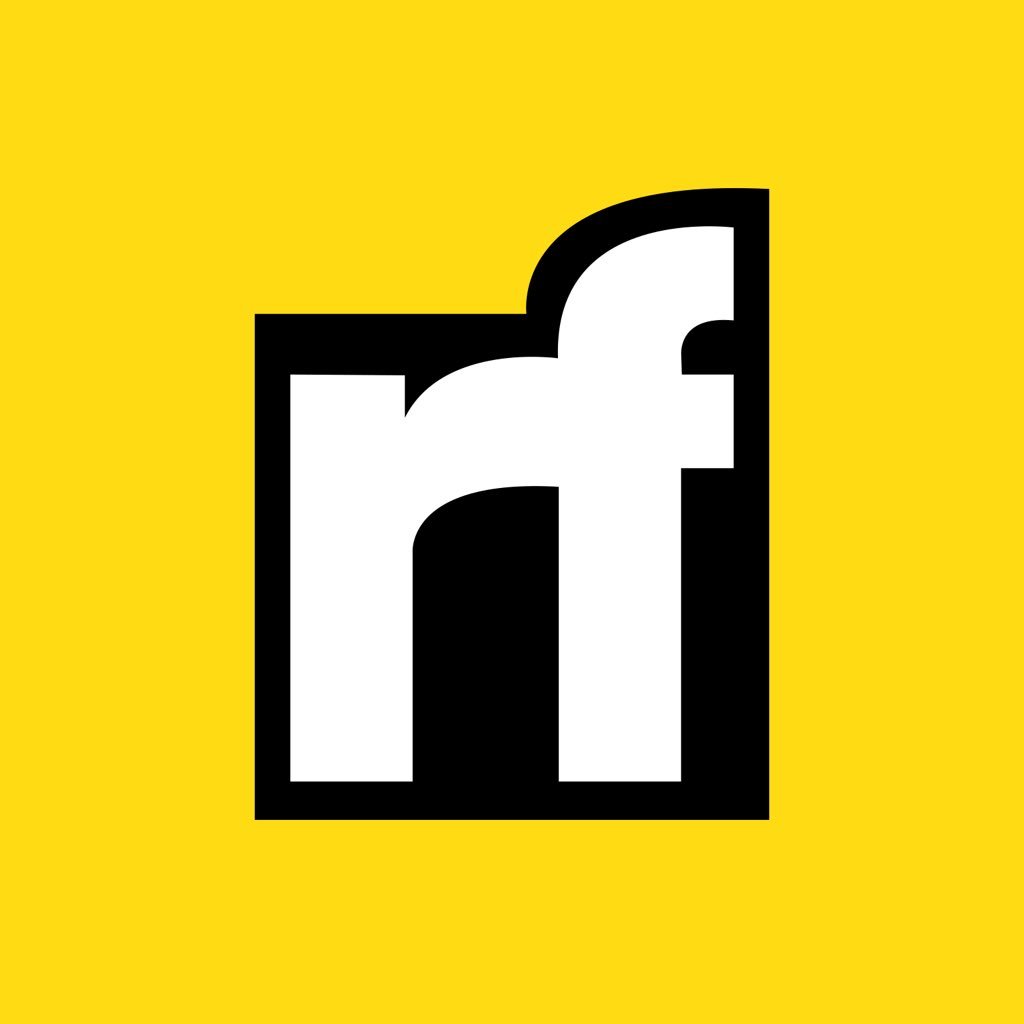
Auto Encoder: How to Ignore the Signal Noise | Sciencx (2024-10-12T22:44:06+00:00) 12 Key Aspects for Assessing the Power of Text-to-Image Models. Retrieved from https://www.scien.cx/2024/10/12/12-key-aspects-for-assessing-the-power-of-text-to-image-models/
Please log in to upload a file.
There are no updates yet.
Click the Upload button above to add an update.