This content originally appeared on HackerNoon and was authored by sshshln
==Conceptual foundations of the phenomenon==
\ Decentralized Science (DeSci) and Artificial Intelligence (AI) are two emerging domains that hold significant potential to transform the way research is conducted, shared, and funded. DeSci leverages blockchain and decentralized protocols to promote open collaboration, transparent funding mechanisms, and equitable rewards for contributors.
\ AI, on the other hand, has revolutionized data processing, predictive modeling, and the automation of complex tasks. When fuse, they form a new phenomenon — let us name it DeScAI, offering a blueprint for a more inclusive, transparent, and efficient scientific enterprise. This article explores the foundations of DeSci, the strengths of AI in science, and the synergistic potential that emerges from their convergence.
1. DeSci: Basics
DeSci heralds a transformative reimagining of how research is carried out, financed, and shared. Harnessing blockchain and other decentralized technologies, DeSci tackles long-standing inefficiencies and inequities within conventional scientific systems. In doing so, it opens new avenues for transparent governance, equitable incentive models, and decentralized data storage — presenting a compelling alternative to the traditional academic status quo.
\ Conventional research frameworks often grapple with systemic roadblocks: expensive paywalls, limited funding avenues, and a peer review process known for its opacity and sluggish pace [Baker, 2016; Begley & Ellis, 2012; Björk et al., 2010; Brembs, 2018; Fang & Casadevall, 2016; Larivière et al., 2015; Piwowar et al., 2018; Romero, 2019; Ioannidis, 2005; Prinz et al., 2011]. These barriers marginalize underfunded scientists, discourage innovation, and restrict broader access to findings. DeSci strives to remedy these challenges through four foundational principles:
🟡 Open Access and Transparency
In a DeSci ecosystem, research outputs — articles, data, and findings — are openly available within permissionless environments. This global accessibility levels the playing field for researchers across diverse socioeconomic contexts. Moreover, blockchain-based storage and distribution eliminate paywalls and subscription fees, fostering broader collaboration and knowledge exchange.
🟡 Fair Compensation and Incentives
Traditional academic structures frequently fail to reward all contributors equitably, particularly data curators, peer reviewers [Trovò & Massari, 2021; Else, 2024], and early-career researchers. DeSci addresses this gap by introducing token-based incentive systems that directly compensate every contributor. By aligning rewards with tangible contributions, these models cultivate a more inclusive scientific community.
🟡 Immutable Records
Distributed ledger technologies help safeguard the authenticity and integrity of scientific outputs. Storing articles, datasets, source code, and related assets on platforms like IPFS or Arweave makes them verifiable and tamper-resistant. This mitigates risks associated with data fabrication, plagiarism, or loss due to centralized storage failures, thereby bolstering the reliability of the scientific record.
🟡 Peer-to-Peer Funding
DeSci’s emphasis on democratizing research extends to funding mechanisms. Scientists can tap into science DAO crowdfunding platforms or solicit direct investment from interested stakeholders, bypassing traditional funding agencies that may be constrained by political or institutional considerations [Bischof et al., 2022; Fantaccini et al, 2024]. This more open, peer-to-peer approach fosters diversity and autonomy in research endeavors.
🟡 Tokenized Research Outputs
IP-NFTs (Intellectual Property Non-Fungible Tokens) revolutionize IP management in DeSci, granting researchers ownership, monetization, and transparent transactions on the blockchain [Bamakan et al., 2022; Shilina, 2023]. Platforms like Molecule, VitaDAO, and AthenaDAO enable fractional ownership and governance over cutting-edge research, while smart contracts automate royalties and simplify collaboration.
\ Fundamentally, DeSci reshapes governance, reward systems, data management, and funding pathways, creating a foundation for a more transparent, equitable, and efficient scientific landscape.
2. The AI Revolution in Science
AI has rapidly evolved into a driving force across numerous scientific and industrial fields, including healthcare, genomics, materials science, and climate modeling [Stevens et al., 2020; Krenn et al., 2022; Xu et al, 2021]. Over the last decade, the convergence of powerful machine learning (ML) algorithms, high-performance computing, and vast datasets has reshaped the way researchers approach complex challenges.
\ Expediting data analysis, automating repetitive tasks, and revealing hidden patterns, AI is accelerating the pace of scientific breakthroughs.
\ ==Key areas where AI has expanded its role include:==
🟡 Processing Large Datasets
In an era of big data, scientific inquiry often involves enormous datasets — ranging from genomic sequences to climate simulations to satellite imagery. AI methods, especially deep learning, excel at detecting correlations and patterns in these datasets, offering novel insights.
\ In genomics, for instance, AI-driven analysis has substantially shortened the time needed to sequence DNA and pinpoint genetic markers tied to various diseases.
🟡 Predicting Outcomes
AI’s predictive capabilities are critical in sectors like drug discovery, where ML models help foresee molecular interactions and potential therapeutic effects. Beyond biomedicine, AI-enabled climate simulations enhance weather forecasting and environmental monitoring, supporting better disaster preparedness and resource allocation. Notably, DeepMind’s AlphaFold transformed protein structure prediction — a feat that had eluded scientists for decades.
🟡 Accelerating Discovery
Modern AI-driven “discovery engines” can automate and optimize key aspects of the scientific method, from generating hypotheses to refining experimental methodologies. In materials science, for example, ML algorithms assist in identifying novel compounds with target properties, thereby speeding up the development of cutting-edge materials and renewable energy innovations.
\ Additionally, OpenAI has created an AI model for longevity science, marking a strategic move into scientific discovery. This specialized system is designed to facilitate the manufacture of stem cells, illustrating how AI can unlock new frontiers in regenerative medicine and other advanced healthcare research areas.
🟡 Enhancing Interdisciplinary Research
AI fosters collaborative research across diverse scientific specialties by providing shared frameworks and analytical tools for data exploration. This synergy has been especially evident at the intersection of neuroscience and computer science, resulting in advancements in brain-computer interfaces and cognitive modeling.
\ Integrating AI technologies into every phase of the research lifecycle, scientists are not only pushing the boundaries of current knowledge but also reimagining how discoveries are made.
3. The Convergence of DeSci and AI: Towards DeScAI
Combining the decentralized infrastructure of blockchain with the computational power and intelligence of advanced AI algorithms, DeScAI emerges as a revolutionary paradigm. This convergence addresses significant limitations in both fields, offering solutions to longstanding challenges in transparency, equity, scalability, and efficiency within the scientific ecosystem. Integrating blockchain-based verification and open data sharing with AI-driven analysis creates a scientific framework that is inclusive, transparent, and participatory.
🟡 An Open-Access Knowledge Reservoir
In the DeScAI paradigm, knowledge isn’t locked behind subscription fees or proprietary platforms. Instead, every experiment, dataset, and publication is stored securely in a distributed ledger. Anyone can view, verify, or contribute findings, and AI agents assist in curating and contextualizing this information, making it easier for researchers everywhere to find what they need.
\ Imagine an emergent “collective memory” that continuously updates as new data streams in — an ever-evolving library where citizens, organizations, and AI systems all act as librarians.
🟡 Shared Global Supercomputing
High-performance computing doesn’t belong solely to tech giants or elite institutions. Instead, millions of individuals and organizations around the world pool their spare processing power into a decentralized “supercomputer.” Researchers tap into this resource to train complex machine learning models — everything from simulating protein folding to improving climate predictions.
\ This global grid is powered by smart incentives: participants receive token rewards proportional to the computational power they contribute. AI orchestrates workload distribution, ensuring tasks are allocated efficiently and ethically.
🟡 Community-Governed Funding
Gone are the days of opaque grant applications and restrictive funding bodies. Scientists propose projects openly to communities of peers and engaged citizens, who vote or stake tokens to support the initiatives they believe in. AI algorithms assist by analyzing proposals, identifying potential overlaps or synergies, and calculating projected impacts.
\ Funding decisions are transparent, traceable, and inclusive; even a lone student with a brilliant idea can secure global backing if the community recognizes the project’s promise.
🟡 Automated and Adaptive Peer Review
Peer review, once plagued by delays and bias, becomes a constructive, collaborative, and rapid process. Research findings are uploaded to a distributed ledger, where AI tools check for data integrity, proper methodology, and potential conflicts of interest.
\ Peer reviewers — who can be anyone with relevant expertise — are rewarded for constructive feedback, while their reputations grow if subsequent studies confirm the validity of their assessments. This continuous feedback loop creates a dynamic research ecosystem, where the community collectively nurtures scientific progress.
🟡Personalized Scientific Discovery
With DeScAI, breakthroughs don’t come exclusively from large labs or specialized institutions. Researchers can customize AI “co-pilots” to sift through troves of data, generating unique hypotheses tailored to their specific questions. For instance, a small team of ecologists might train an AI model on planetary data to study local biodiversity, while another group could focus on mapping viral mutations.
\ Because all datasets and computing resources are decentralized, any researcher can access or refine these models, turning individual curiosities into global innovations.
🟡 Ethical and Equitable Data Economies
Data is the raw material fueling AI advancements, but it belongs to the people who generate it — patients, citizens, volunteers, or entire communities. Rather than being exploited by centralized entities, individuals and groups maintain ownership of their data and can choose how, when, and under what conditions it’s shared.
\ Whenever data is used to train models, contributors receive fair compensation or governance tokens. AI helps enforce usage restrictions and anonymization policies, ensuring data is both protected and utilized responsibly.
🟡 Holistic Collaboration Beyond Borders
Scientific progress is no longer limited by geographic, institutional, or economic boundaries. A biologist in Lagos can seamlessly collaborate with a roboticist in Tokyo, aided by AI-driven translation tools and a shared dataset stored on decentralized infrastructure. These cross-disciplinary “fusion teams” form spontaneously around global challenges — everything from creating sustainable energy solutions to eradicating neglected tropical diseases. Each discovery feeds back into the worldwide knowledge reservoir, sparking new questions and innovations in a virtuous cycle.
4. Episteme: The Future of Decentralized AI-Driven Science
At Episteme, we merge the core principles of DeSci — openness, equity, and community-driven collaboration — with the cutting-edge capabilities of AI. This fusion, DeScAI as we name it, propels our vision of creating a global, gamified platform for forecasting scientific breakthroughs.
Decentralized Infrastructure Meets AI-Powered Insights:
🟡 Immutable, transparent research
Episteme’s platform relies on decentralized ledger technology to host research, data, and verification records. This blockchain-based foundation guarantees immutability and transparency, ensuring that bets on scientific hypotheses are secure and tamper-proof.
\ 🟡 AI-driven integrity checks
AI algorithms constantly scan and validate data entries, detect inconsistencies, and assess the credibility of participants’ predictions. This automated oversight reduces the likelihood of fraudulent activity and fosters a more trustworthy environment for scientific betting.
Tokenized Prediction Markets Enhanced by AI:
🟡 Engaging, gamified bets
By pairing tokenomics with gamification, Episteme incentivizes public participation in the future of science. Participants “bet” on whether a scientific hypothesis or milestone will be achieved, creating a dynamic market that makes science both interactive and accessible. Prediction markets not only draw public interest but also engage a broader audience in scientific validation.
\ 🟡 AI for real-time accuracy and resolution
AI curates incoming data from various sources — published studies, expert analyses, and other updates — to adjust prediction market odds in real-time. This ensures that markets remain up-to-date, informative, and responsive to breakthroughs as they unfold. Beyond this, AI also plays a central role in resolving markets by analyzing verified data and determining outcomes with transparency and precision.
\ Leveraging trusted data sources and advanced algorithms, AI ensures the resolution process is fast, unbiased, and trustless, providing participants with a reliable framework for engaging in scientific predictions.
The Future of DeScAI at Episteme
Blending decentralized governance with AI-powered prediction markets, Episteme reimagines the creation, validation, and monetization of scientific knowledge. In this new paradigm:
\
- AI provides real-time insights and resolves bets, guiding participants through the complexities of scientific discovery.
- Tokenomics ensures that data contributors, researchers, and bettors share collective success.
- The community steers research direction through transparent wagers and funding decisions.
\ Episteme’s DeScAI approach sets the stage for an era of open, incentivized, and community-driven science — making breakthroughs more achievable, engaging, and equitable than ever before. Harnessing an advanced AI stack alongside decentralized principles, Episteme redefines the scientific landscape, igniting a future where everyone can contribute to, learn from, and benefit directly from the world’s most pressing discoveries.
Conclusion
The synergy of decentralized science and artificial intelligence — DeScAI — signals a groundbreaking shift in how research is conducted, shared, and enriched. “Now is the time to understand more, so that we may fear less,” Marie Curie once observed, a sentiment echoing through DeScAI’s open and transparent approach.
\ Blockchain’s data integrity merges with AI’s real-time analysis to dismantle barriers in computation, funding, and peer review, transforming the scientific enterprise into an equitable, collaborative environment.
\ Rethinking incentives, automating verification, and exploring uncharted territories of discovery pave the way for a future where knowledge remains a collective right, fueling boundless innovation and human progress.
References
- Baker, M. (2016). 1,500 scientists lift the lid on reproducibility. Nature, 533(7604), 452–454. https://doi.org/10.1038/533452a
- Bamakan, S. M., Nezhadsistani, N., Bodaghi, O., & Qu, Q. (2022). Patents and intellectual property assets as non-fungible tokens; key technologies and challenges. Scientific Reports, 12(1), 1–13. https://doi.org/10.1038/s41598-022-05920-6
- Bartling, S., & Fecher, B. (2017). Blockchain for science and knowledge creation: Promises and potentials of distributed ledger technology in knowledge exchange. Medium. https://doi.org/10.31235/osf.io/5ujd8
- Björk, B.-C., Welling, P., Laakso, M., Majlender, P., Hedlund, T., & Guðnason, G. (2010). Open Access to the scientific journal literature: Situation 2009. PLoS ONE, 5(6), e11273.
- Bischof, E., Botezatu, A., Jakimov, S., Suharenko, I., Ostrovski, A., Verbitsky, A., et al. (2022). Longevity foundation: Perspective on decentralized autonomous organizations for special-purpose financing. IEEE Access, 10, 33048–33058. https://doi.org/10.1109/ACCESS.2022.3161392
- Else, H. (2024). ‘Getting paid to review is justice’: Journal pays peer reviewers in cryptocurrency. Nature. https://doi.org/10.1038/d41586-024-04027-4
- Fang, F. C., & Casadevall, A. (2012). Reforming science: Structural reforms. Infection and Immunity, 80(3), 897–901.
- Fantaccini, S., Grassi, L., & Rampoldi, A. (2024). The potential of DAOs for funding and collaborative development in the life sciences. Nature Biotechnology, 42(4), 555–562. https://doi.org/10.1038/s41587-024-02189-0
- Ioannidis, J. P. A. (2005). Why most published research findings are false. PLoS Medicine, 2(8), e124.
- Larivière, V., Haustein, S., & Mongeon, P. (2015). The oligopoly of academic publishers in the digital era. PLoS ONE, 10(6), e0127502. https://doi.org/10.1371/journal.pone.0127502
- Krenn, M., Pollice, R., Guo, S. Y., Aldeghi, M., Cervera-Lierta, A., Friederich, P., … & Aspuru-Guzik, A. (2022). On scientific understanding with artificial intelligence. Nature Reviews Physics, 4(12), 761–769.
- Piwowar, H., Priem, J., Orr, R., et al. (2018). The state of OA: A large-scale analysis of the prevalence and impact of Open Access articles. PeerJ, 6, e4375.
- Polanyi, M. (1962). The republic of science: Its political and economic theory. Minerva, 1(1), 54–73.
- Romero, F. (2019). Philosophy of science and reproducibility crisis. Synthese, 196(8), 3067–3086.
- Stevens, R., Taylor, V., Nichols, J., Maccabe, A. B., Yelick, K., & Brown, D. (2020). Ai for science: Report on the department of energy (doe) town halls on artificial intelligence (ai) for science (No. ANL-20/17). Argonne National Lab.(ANL), Argonne, IL (United States).
- Xu, Y., Liu, X., Cao, X., Huang, C., Liu, E., Qian, S., … & Zhang, J. (2021). Artificial intelligence: A powerful paradigm for scientific research. The Innovation, 2(4).
\
This content originally appeared on HackerNoon and was authored by sshshln
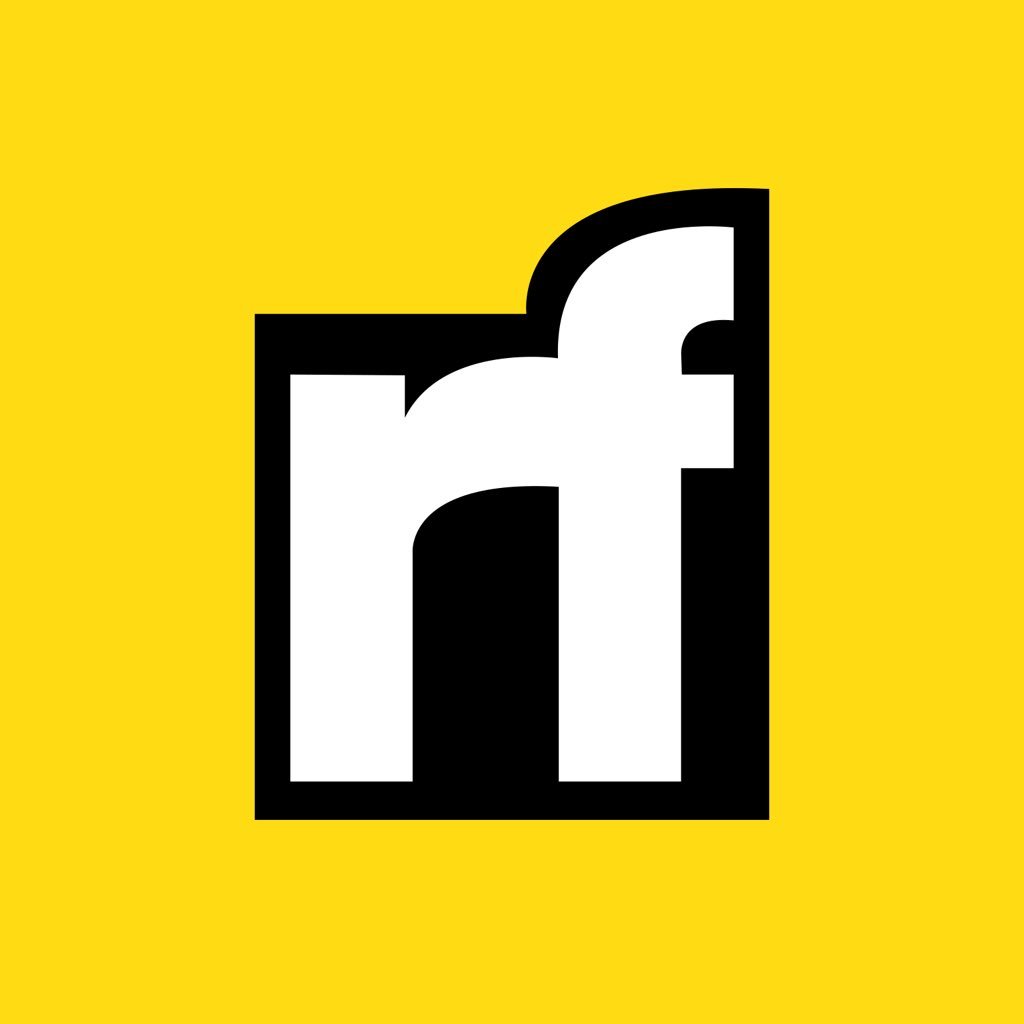
sshshln | Sciencx (2025-01-22T00:07:30+00:00) DeScAI: When Decentralized Science Meets Artificial Intelligence. Retrieved from https://www.scien.cx/2025/01/22/descai-when-decentralized-science-meets-artificial-intelligence/
Please log in to upload a file.
There are no updates yet.
Click the Upload button above to add an update.