This content originally appeared on HackerNoon and was authored by Seismology
:::info Authors:
(1) Cody Rucker, Department of Computer Science, University of Oregon and Corresponding author;
(2) Brittany A. Erickson, Department of Computer Science, University of Oregon and Department of Earth Sciences, University of Oregon.
:::
Table of Links
Abstract and 1. Context and Motivation
- Physics-Informed Deep Learning Framework
- Learning Problems for Earthquakes on Rate-and-State Faults
- 2D Verification, Validation and Applications
- Summary and Future Work and References
4. 2D Verification, Validation and Applications
When computational methods for physical problems are used to address science questions, verification is an essential first step to ensure credible results [20, 12]. While validation with observational data is the focus of future work, we must first verify that our physics-informed deep learning framework is able to solve both forward and inverse problems to reasonable accuracy.
4.1. Verification with the Method of Manufactured Solutions
\
\
\
\
\
\
\
\
:::info This paper is available on arxiv under CC BY 4.0 DEED license.
:::
\
This content originally appeared on HackerNoon and was authored by Seismology
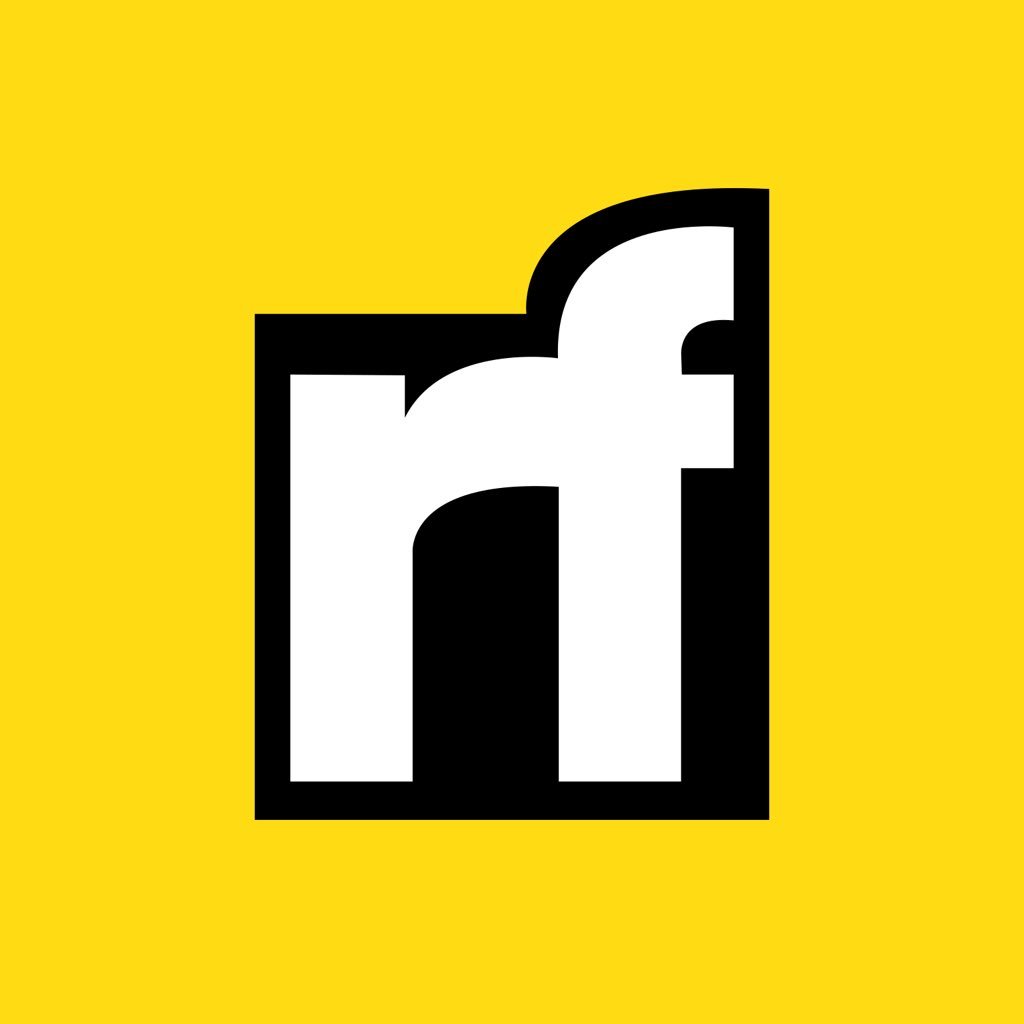
Seismology | Sciencx (2024-08-02T15:00:14+00:00) Integrating Physics-Informed Neural Networks for Earthquake Modeling: 2D Verification, Applications. Retrieved from https://www.scien.cx/2024/08/02/integrating-physics-informed-neural-networks-for-earthquake-modeling-2d-verification-applications/
Please log in to upload a file.
There are no updates yet.
Click the Upload button above to add an update.