This content originally appeared on DEV Community and was authored by Super Kai (Kazuya Ito)
*Memos:
RMSProp() can do gradient descent by automatically adapting learning rate to parameters as shown below:
*Memos:
- The 1st argument for initialization is
params
(Required-Type:generator
). - The 2nd argument for initialization is
lr
(Optional-Default:0.01
-Type:int
orfloat
). *It must be0 <= x
. - The 3rd argument for initialization is
alpha
(Optional-Default:0.99
-Type:int
orfloat
). *It must be0 <= x
. - The 4th argument for initialization is
eps
(Optional-Default:1e-08
-Type:int
orfloat
). - The 5th argument for initialization is
weight_decay
(Optional-Default:0
-Type:int
orfloat
). *It must be0 <= x
. - The 6th argument for initialization is
momentum
(Optional-Default:0
-Type:int
orfloat
). *It must be0 <= x
. - The 7th argument for initialization is
centered
(Optional-Default:False
-Type:bool
). - The 8th(CUDA) argument for initialization is
capturable
(Optional-Default:False
-Type:bool
). *Setting it on CUDA(GPU) works while setting it on CPU gets error. - The 8th(CPU) or 9th(CUDA) argument for initialization is
foreach
(Optional-Default:None
-Type:bool
). - The 9th(CPU) or 10th(CUDA) argument for initialization is
maximize
(Optional-Default:False
-Type:bool
). - The 10th(CPU) or 11th(CUDA) argument for initialization is
differentiable
(Optional-Default:False
-Type:bool
). - step() can update parameters.
- zero_grad() can reset gradients.
from torch import nn
from torch import optim
class MyModel(nn.Module):
def __init__(self):
super().__init__()
self.linear_layer = nn.Linear(in_features=4, out_features=5)
def forward(self, x):
return self.linear_layer(x)
mymodel = MyModel()
rmsprop = optim.RMSprop(params=mymodel.parameters())
rmsprop
# RMSprop (
# Parameter Group 0
# alpha: 0.99
# centered: False
# differentiable: False
# eps: 1e-08
# foreach: None
# lr: 0.01
# maximize: False
# momentum: 0
# weight_decay: 0
# )
rmsprop.state_dict()
# {'state': {},
# 'param_groups': [{'lr': 0.01,
# 'momentum': 0,
# 'alpha': 0.99,
# 'eps': 1e-08,
# 'centered': False,
# 'weight_decay': 0,
# 'foreach': None,
# 'maximize': False,
# 'differentiable': False,
# 'params': [0, 1]}]}
rmsprop.step()
rmsprop.zero_grad()
# None
# This is for CPU without `capturable`
rmsprop = optim.RMSprop(params=mymodel.parameters(), lr=0.01, alpha=0.99,
eps=1e-08, weight_decay=0, momentum=0,
centered=False, foreach=None,
maximize=False, differentiable=False)
rmsprop
# RMSprop (
# Parameter Group 0
# alpha: 0.99
# centered: False
# differentiable: False
# eps: 1e-08
# foreach: None
# lr: 0.01
# maximize: False
# momentum: 0
# weight_decay: 0
# )
# This is for CUDA(GPU) with `capturable`
rmsprop = optim.RMSprop(params=mymodel.parameters(), lr=0.01, alpha=0.99,
eps=1e-08, weight_decay=0, momentum=0,
centered=False, capturable=False, foreach=None,
maximize=False, differentiable=False)
rmsprop
# RMSprop (
# Parameter Group 0
# alpha: 0.99
# capturable: False
# centered: False
# differentiable: False
# eps: 1e-08
# foreach: None
# lr: 0.01
# maximize: False
# momentum: 0
# weight_decay: 0
# )
This content originally appeared on DEV Community and was authored by Super Kai (Kazuya Ito)
Print
Share
Comment
Cite
Upload
Translate
Updates
There are no updates yet.
Click the Upload button above to add an update.
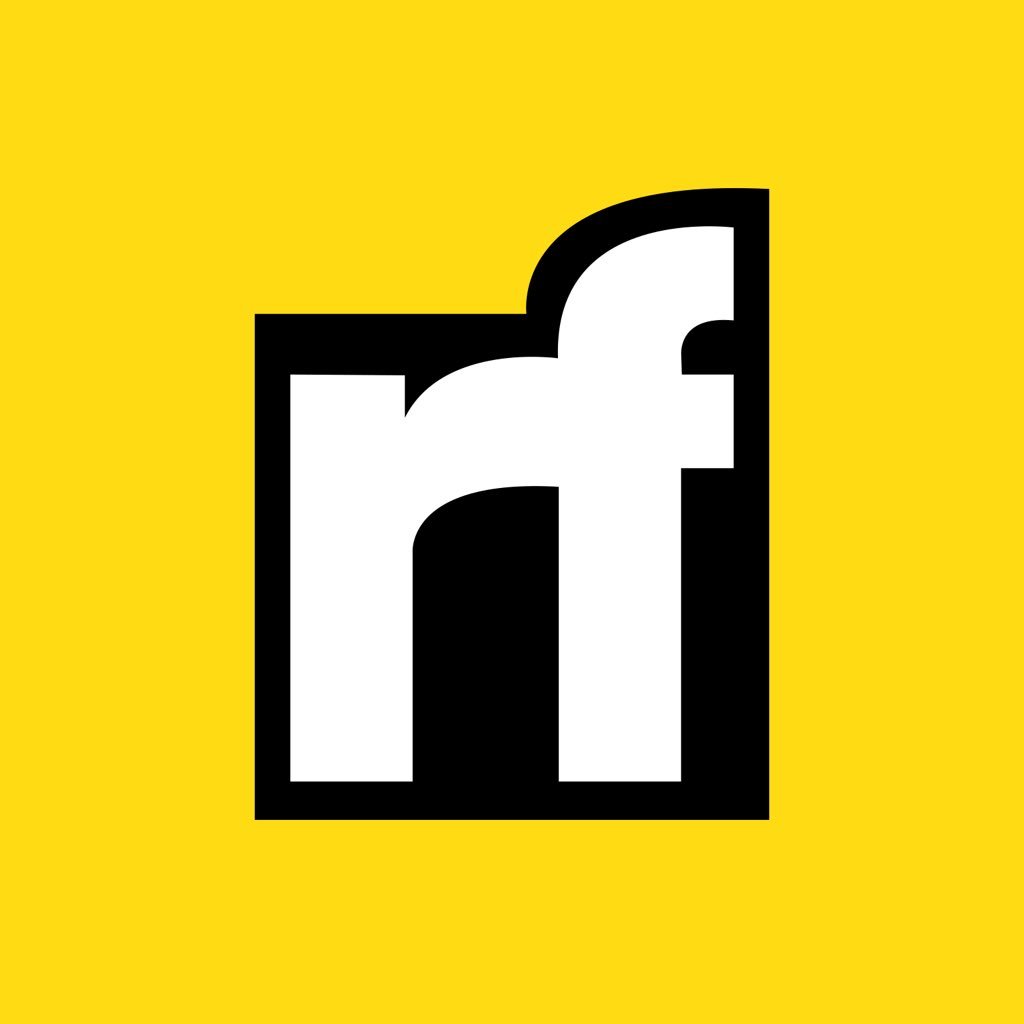
APA
MLA
Super Kai (Kazuya Ito) | Sciencx (2024-10-05T01:34:13+00:00) RMSProp in PyTorch. Retrieved from https://www.scien.cx/2024/10/05/rmsprop-in-pytorch/
" » RMSProp in PyTorch." Super Kai (Kazuya Ito) | Sciencx - Saturday October 5, 2024, https://www.scien.cx/2024/10/05/rmsprop-in-pytorch/
HARVARDSuper Kai (Kazuya Ito) | Sciencx Saturday October 5, 2024 » RMSProp in PyTorch., viewed ,<https://www.scien.cx/2024/10/05/rmsprop-in-pytorch/>
VANCOUVERSuper Kai (Kazuya Ito) | Sciencx - » RMSProp in PyTorch. [Internet]. [Accessed ]. Available from: https://www.scien.cx/2024/10/05/rmsprop-in-pytorch/
CHICAGO" » RMSProp in PyTorch." Super Kai (Kazuya Ito) | Sciencx - Accessed . https://www.scien.cx/2024/10/05/rmsprop-in-pytorch/
IEEE" » RMSProp in PyTorch." Super Kai (Kazuya Ito) | Sciencx [Online]. Available: https://www.scien.cx/2024/10/05/rmsprop-in-pytorch/. [Accessed: ]
rf:citation » RMSProp in PyTorch | Super Kai (Kazuya Ito) | Sciencx | https://www.scien.cx/2024/10/05/rmsprop-in-pytorch/ |
Please log in to upload a file.
There are no updates yet.
Click the Upload button above to add an update.