This content originally appeared on HackerNoon and was authored by Economic Hedging Technology
Table of Links
Introduction
CONCLUSIONS
In summary, this article fills a void in the DRL hedging field, which primarily focuses on European options, by introducing the application of DRL agents to hedge American put options. The study utilizes the DDPG algorithm for this purpose. Notably, while the state variables— comprising the asset price, current holding, and time-to-maturity—conform to existing literature, a novelty of this research lies in a unique reward function. This reward function accounts for both the negative absolute difference between the option value and the underlying asset position value and incorporates a quadratic penalty for transaction costs. The first round of experiments uses GBM asset paths for both training and testing, and initial results show that under the presence of transaction costs, the DRL agent outperforms the BS Delta and binomial tree hedging strategies. Another preliminary result of this work is that when the hedging agent underestimates the volatility, the DRL agent once again outperforms the BS Delta and Binomial strategies.
\ This article extends the findings of the GBM results by training DRL agents using asset paths generated through a stochastic volatility model. Stochastic volatility model parameters are calibrated to each symbol by using 80 options from eight symbols, featuring two maturities and five strikes. In contrast to the GBM approach, where a binomial tree interpolation provides option prices at each time step, option prices for stochastic volatility experiments are computed using a Chebyshev interpolation method, which eliminates the requirement to compute average payoffs from MC simulations up to maturity or early exercise at each training step. The outcomes reveal that the DRL agent not only outperforms the BS Delta strategy when tested with paths generated from the same stochastic volatility model used in training, but the DRL agent also exhibits superior hedging performance, on average, as compared to BS Delta when testing with the realized underlying assets between the option sale date and maturity. Finally, note that as the Chebyshev pricing method is model agnostic, this work contributes a general framework that may be extended to train DRL agents for any desired underlying asset process.
\ These results imply that DRL agents may be employed in an empirical setting to effectively hedge American put options by calibrating the training process to listed option data. A recommended future direction for more optimal results would be to retrain the agent more frequently as new option data becomes available. Further, a future study that examines the effect of training hyperparameters is recommended to aid practitioners in starting to train their own DRL agents.
\
:::info Authors:
(1) Reilly Pickard, Department of Mechanical and Industrial Engineering, University of Toronto, Toronto, ON M5S 3G8, Canada (reilly.pickard@mail.utoronto.ca);
(2) Finn Wredenhagen, Ernst & Young LLP, Toronto, ON, M5H 0B3, Canada;
(3) Julio DeJesus, Ernst & Young LLP, Toronto, ON, M5H 0B3, Canada;
(4) Mario Schlener, Ernst & Young LLP, Toronto, ON, M5H 0B3, Canada;
(5) Yuri Lawryshyn, Department of Chemical Engineering and Applied Chemistry, University of Toronto, Toronto, ON M5S 3E5, Canada.
:::
:::info This paper is available on arxiv under CC BY-NC-SA 4.0 Deed (Attribution-Noncommercial-Sharelike 4.0 International) license.
:::
\
This content originally appeared on HackerNoon and was authored by Economic Hedging Technology
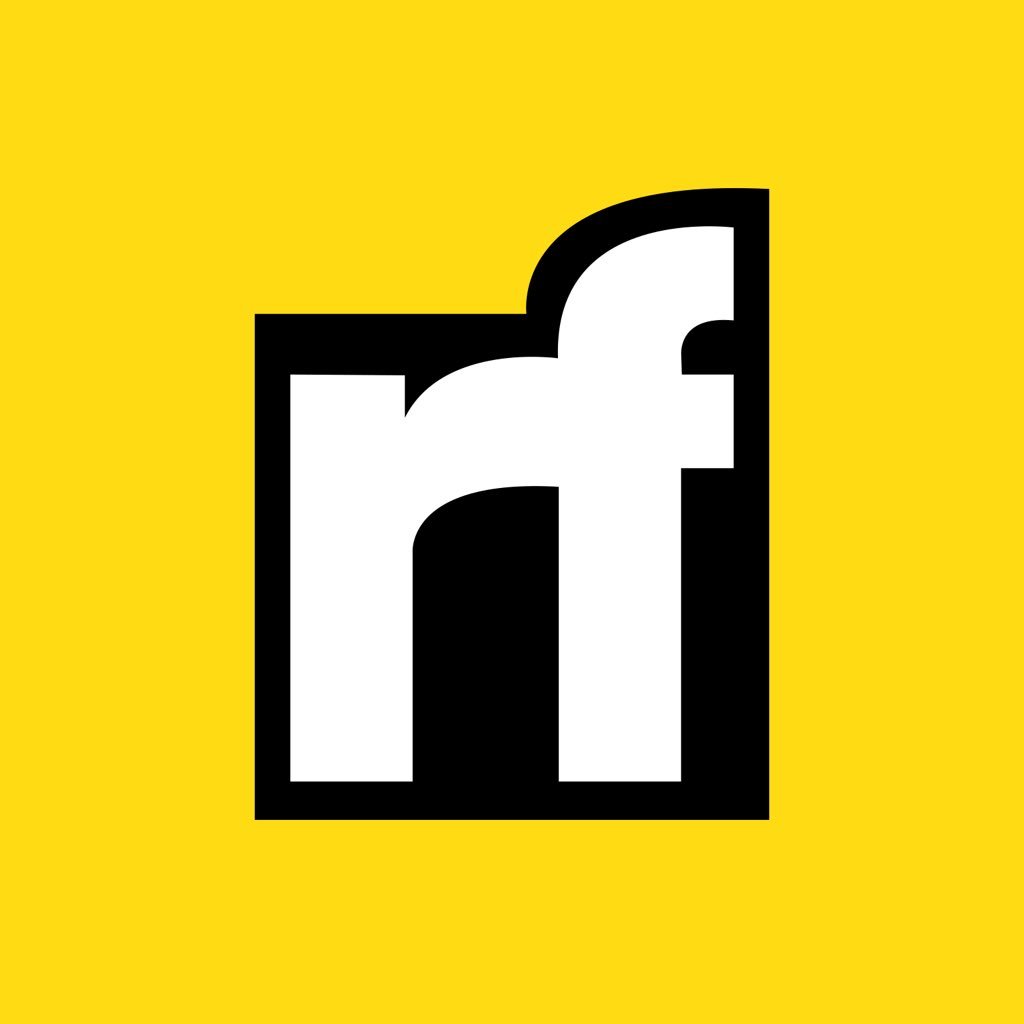
Economic Hedging Technology | Sciencx (2024-10-30T17:45:13+00:00) Advancements in Deep Reinforcement Learning for Hedging American Put Options. Retrieved from https://www.scien.cx/2024/10/30/advancements-in-deep-reinforcement-learning-for-hedging-american-put-options/
Please log in to upload a file.
There are no updates yet.
Click the Upload button above to add an update.