This content originally appeared on HackerNoon and was authored by Model Tuning
:::info Author:
(1) Andrew J. Peterson, University of Poitiers (andrew.peterson@univ-poitiers.fr).
:::
Table of Links
The media, filter bubbles and echo chambers
Network effects and Information Cascades
\ Appendix
\
Related Work
Technology has long affected how we access knowledge, raising concerns about its impact on the transmission and creation of knowledge. Yeh Meng-te, for example, argued in the twelfth century that the rise of books led to a decline in the practice of memorizing and collating texts that contributed to a decline of scholarship and the repetition of errors (Cherniack, 1994, p.48-49). Even earlier, a discussion in Plato’s Phaedrus considers whether the transition from oral tradition to reading texts was harmful to memory, reflection and wisdom (Hackforth, 1972).
\ We focus on recent work on the role of digital platforms and social interactions, and mention only in passing the literature on historical innovations and knowledge (e.g. Ong, 2013; Mokyr, 2011; Havelock, 2019), and the vast literature on the printing press (e.g. Dittmar, 2011; Eisenstein, 1980). Like other media transitions before it (Wu, 2011), the rise of internet search algorithms and of social media raised concerns about the nature and distribution of information people are exposed to, and the downstream effects on attitudes and political polarization (Cinelli et al., 2021; Barbera´, 2020).
\ The following section considers research on the impact of recommendation algorithms and self-selection on social media, and how this might generate distorted and polarizing opinions, as an analogy for understanding the transformation brought about by reliance on AI. We consider game theoretic models of information cascades as an alternative model for failure in social learning, in which the public to fails to update rationally on individuals’ private signals. Next, we review the main findings of network analysis on the flow of information in social media, which also identify mechanisms which distort knowledge formation. We then examine the specific nature of generative AI algorithms, focusing on the problem of model collapse and known biases in AI outputs.
\
The media, filter bubbles and echo chambers
A common critique of social media is that they allow users to select in to “echo chambers” (specific communities or communication practices) in which they are exposed to only a narrow range of topics or perspectives. For example, instead of consulting the “mainstream” news where a centrist and relatively balanced perspective is provided, users are exposed to selective content that echoes pre-existing beliefs. In the ideological version of the echo-chamber hypothesis, individuals within a latent ideological space (for example a one-dimensional left-right spectrum), are exposed to peers and content with ideologically-similar views. If so, their beliefs are reinforced socially and by a generalization from their bounded observations, leading to political polarization (Cinus et al., 2022; Jamieson and Cappella, 2008; Pariser, 2011). \n
A simple model for this assumes homophily within in a network growth model, in which similar individuals chose to interact. Implicitly the approach presumes that this is common on social media but not common within traditional media, which for technological reasons were constrained to provide the same content across a broad population with possibly heterogeneous preferences.[1] This general dynamic may hold even if traditional media and newspapers were themselves dynamic systems interacting with their consumers, markets and advertisers, and themselves adapting their message to specific communities and preferences (e.g. Angelucci, Cage, and ´ Sinkinson, forthcoming; Cage´, 2020; Boone, Carroll, and van Witteloostuijn, 2002). \n
The second main line of analysis focuses on “filter bubbles,” whereby the content to which users are exposed is selected based on a recommendation system. Jiang et al. (2019) model this as a dynamic process between a user’s evolving interests and behavior (such as clicking a link, video, or text) and a recommender system which aims to maximize expected utility for the user. In their reinforcement learning-inspired framework, the aim is for the user to explore the space of items or topics without the algorithm assigning degenerate (extremely high or zero) probabilities to these items. As above, a key concern is the political or ideological content of recommendations their relation to polarization (Keijzer and Mas¨ , 2022). In a more recent twist, Sharma, Liao, and Xiao (2024) find that LLM-powered search may generate more selective exposure bias and polarization by reinforcing pre-existing opinions based on finer-grained clues in the user’s queries.
\ Particularly relevant for our context is the issue of “popularity bias” in recommender systems, in which a small subset of content receives wide exposure while users (distributed based on some long-tailed distribution, like the topics) from smaller groups or with rare preferences are marginalized. On the one hand, users may desire to be exposed to popular content, for example to understand trending ideas or fashions. But overly favoring popular items can lead to user disengagement because it neglects their unique interests, lacks variety, etc. (e.g. Klug et al., 2021). Recommendation systems are often biased in the sense that even when a subset of users wants to get access to non-popular items, they receive few or no such recommendations (Abdollahpouri et al., 2021). A number of approaches have been suggested to counteract this tendency (e.g. Lin et al., 2022; Gao et al., 2023). \n
The problem of popularity bias is ironic given that one of the unique contributions of the internet was its ability to provide access to long-tailed products and services that were previously ignored or inaccessible (Brynjolfsson et al., 2006; Brynjolfsson, Hu, and Smith, 2003). By extension, we would expect social media and the internet to make possible a more diverse and rich informational environment. The role of self-selection into communities and recommendation algorithms provides a explanation for why this might not be the case. In the next section we consider a more general set of models that examine information flow within networks and the idea of information cascades. \n
:::info This paper is available on arxiv under CC BY-NC-SA 4.0 DEED license.
:::
[1] The reality is as usual more complex. For example, in the post-war era, the concern was almost the inverse- the fear that the few channels that were possible with television led to ‘homogenization.’ There are also other dynamics at play than technological constraints. For example, in contrast to TV, the 1950s and 1960s saw a proliferation of more diverse and local radio stations, some catering to ethnic minorities and musical tastes outside the mainstream. The ‘payola’ scandals, however, led to regulations that shifted content decisions from diverse DJs to centralized music directors (Douglas, 2002).
This content originally appeared on HackerNoon and was authored by Model Tuning
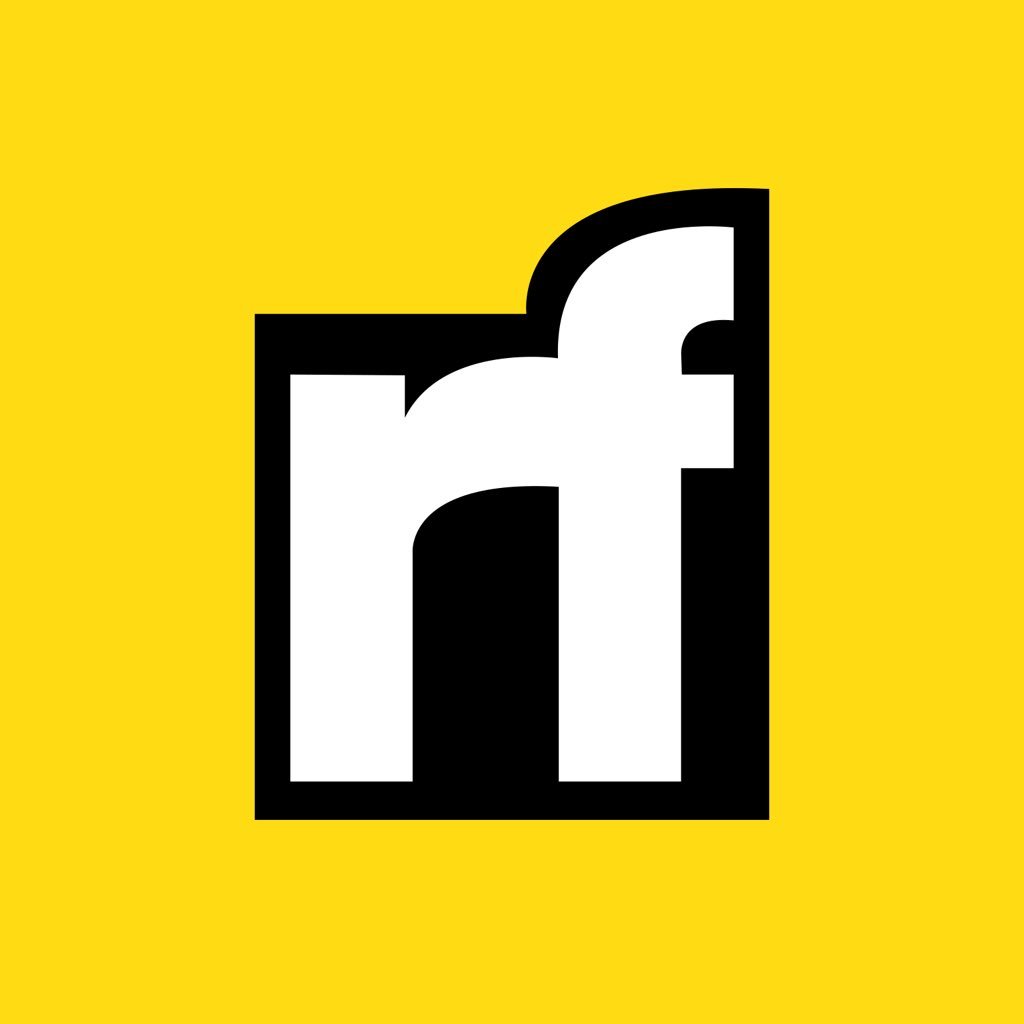
Model Tuning | Sciencx (2025-02-17T21:29:00+00:00) How AI and Social Media Shape Knowledge Through Echo Chambers and Filter Bubbles. Retrieved from https://www.scien.cx/2025/02/17/how-ai-and-social-media-shape-knowledge-through-echo-chambers-and-filter-bubbles/
Please log in to upload a file.
There are no updates yet.
Click the Upload button above to add an update.